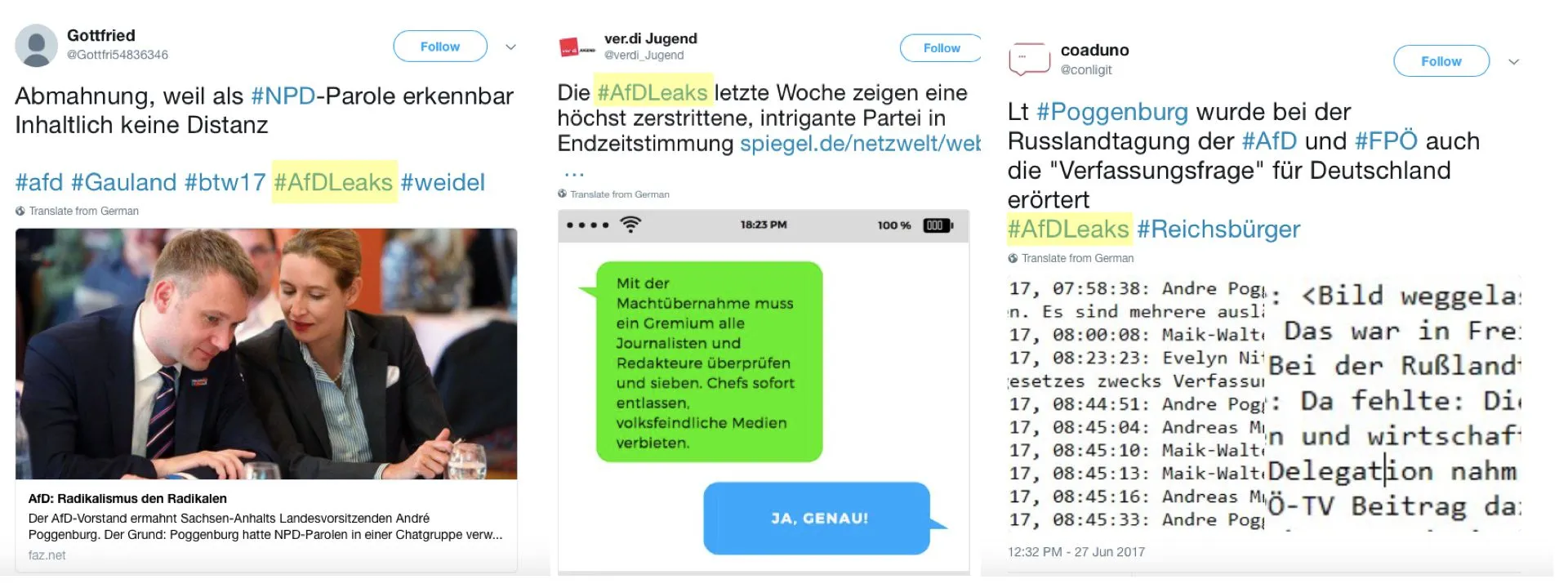
BANNER: Some of the tweets to use the #AfDLeaks hashtag. (Source: @Gottfri54836346 / @verdi_Jugend / @conligit / Twitter)
On June 19, left-wing German website linksunten.de posted online a collection of leaked WhatsApp conversations purporting to be between members of the anti-migrant Alternative für Deutschland (AfD) party.
The leak was politically explosive, as it seemed to show the AfD’s leader of the state of Sachsen-Anhalt (Saxony-Anhalt), André Poggenburg, using the phrase “Germany for the Germans,” a slogan linked to the neo-Nazi movement. According to multiple media reports, Poggenburg confirmed the veracity of the leaked chats and stood by his comments.
#Poggenburg steht zu "Deutschland den Deutschen" – und lehnt Verantwortung für Mitglieder seines Landesverbands ab. #AfDLeaks (1/2) pic.twitter.com/SNtA5n0Rvu
— Lars Wienand (@LarsWienand) June 20, 2017
Soon after the document dump, Twitter users began circulating the hashtag #AfDLeaks, which was used before in other contexts in connection with the incident. According to the online research account @politwi, the hashtag made it into the top political trends by the end of that day:
Politische Top-Themen 20.06. 0 Uhr https://t.co/tzkdKYyYPb
1. #brussels
2. #brüssel
3. #afdleaks
4. #terror
5. #g20 pic.twitter.com/N60bORpSkh— politwi (@politwi) June 20, 2017
The pattern of a political hashtag making it into the trending lists is familiar from other incidents, notably #MacronLeaks and various hashtag drives launched by supporters of the nationalist leader Marine Le Pen in France. On this occasion, however, the hashtag was primarily driven by accounts on from the left of the political spectrum, rather than the right.
It is therefore worth comparing the progress of the #AfDLeaks hashtag with other political hashtag campaigns, to assess the degree to which different political actors use different amplification techniques.
Small and slow
The first thing to note is the small scale of the #AfDLeaks operation. According to a machine scan of all posts on the subject from June 19, the hashtag had generated 3,144 tweets by June 26. This is orders of magnitude lower than #MacronLeaks, which recorded almost 47,000 tweets in under four hours after its launch.
It is also orders of magnitude below the hashtag campaigns that supported leader Marine Le Pen in the French presidential election, which regularly garnered between 20,000 and 40,000 tweets in an hour.
The hashtag was also slow to gain attention. It was used just 19 times on June 19, the day of the leak, and 190 times the following day. It did not pick up until June 21, when it was tweeted 1,365 times that day. The usage spiked again on June 22, then tailed off and dropped below 100 tweets a day by June 25.
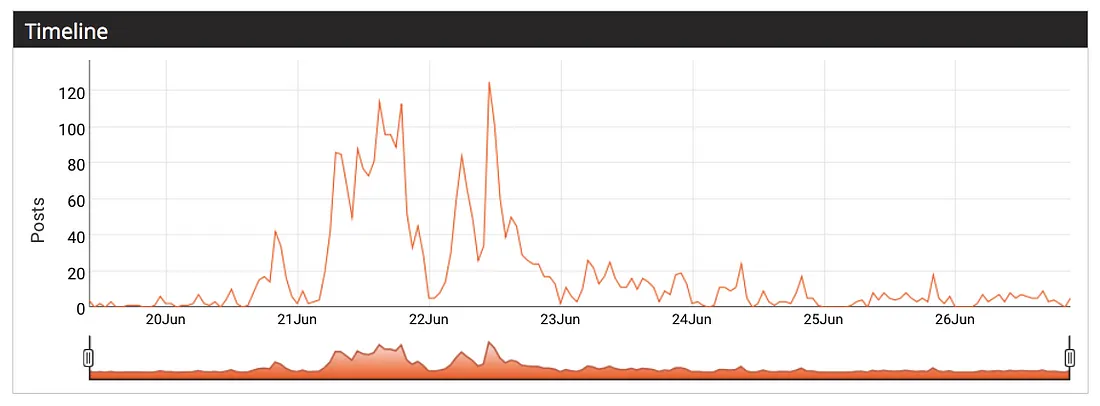
This, again, is markedly different from massive hashtag operations like those launched by the French far-right, which showed a very rapid initial growth and then a steady decline.
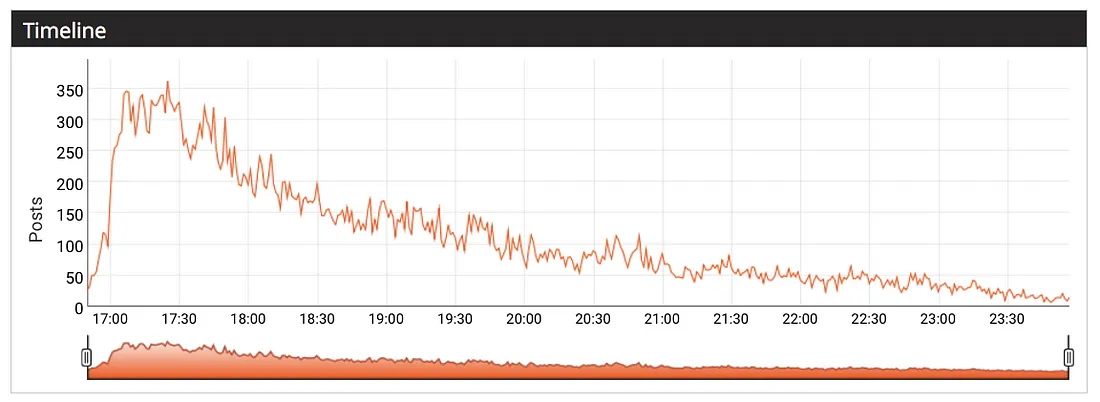
A third difference lies in the intensity with which individual accounts used the hashtag, measured as the average number of tweets generated per user. This indicator can be used to assess whether a small but dedicated group drives a particular hashtag, or whether a larger group of casual and un-coordinated users does so.
In general, the higher the average number of tweets per user, the higher the likelihood that the hashtag drove a coordinated campaign. A high average also suggests that the traffic was amplified by automated “bot” accounts, which post dozens or hundreds of tweets in a short time.
The campaign around #LaFranceVoteMarine, launched on February 26, saw 47,000 tweets posted by 8,860 users, with an average of 5.3 tweets per user. This is a relatively high figure; closer analysis revealed a number of highly-active accounts, apparently automated, amplifying the hashtag.
The early hours of #MacronLeaks saw 47,000 tweets posted by 14,118 users, with an average of 3.3 tweets per user. This is a mid-range figure; the traffic was amplified by some automated accounts, but to a lesser degree.
By contrast, #AfDLeaks saw 3,144 tweets posted by 1,905 users, for an average of 1.6 tweets per user, a relatively low figure.
The low overall volume of tweets, the gradual increase in traffic, and the low degree of engagement per user all suggest that #AfDLeaks spread organically, without significant offline coordination and without the use of automated bot amplifiers.
Ubiquitous bots
Nevertheless, #AfDLeaks was boosted by some accounts with suspiciously bot-like tendencies.
The most obvious potential bot used was the one with the screen name “Destiny Shelton,” and the Twitter handle @Destiny73928407. On June 26, this account retweeted the most popular tweet on #AfDLeaks, from an account called @GoaGoaZwerg:
Several things about this account are suspect. It was created on June 22, 2017. According to a machine scan of its tweets, it posted twice that day, 51 times on June 24, 99 times on June 25, and then fell silent. With the exception of its posts on June 22, every single one was a retweet.
The account’s avatar is that of fitness model Helga Lovekaty, who regularly posts images of herself on her Instagram feed.
These include the picture that “Destiny Shelton” uses as its avatar:
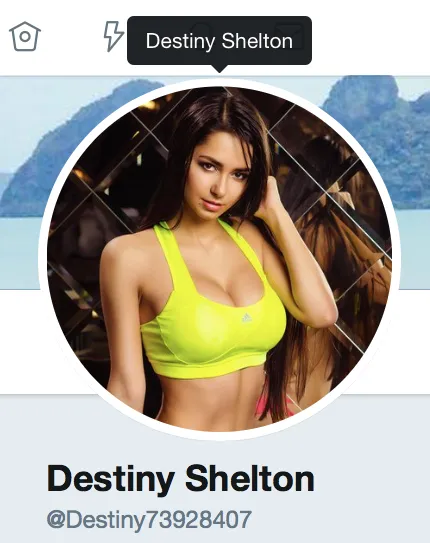
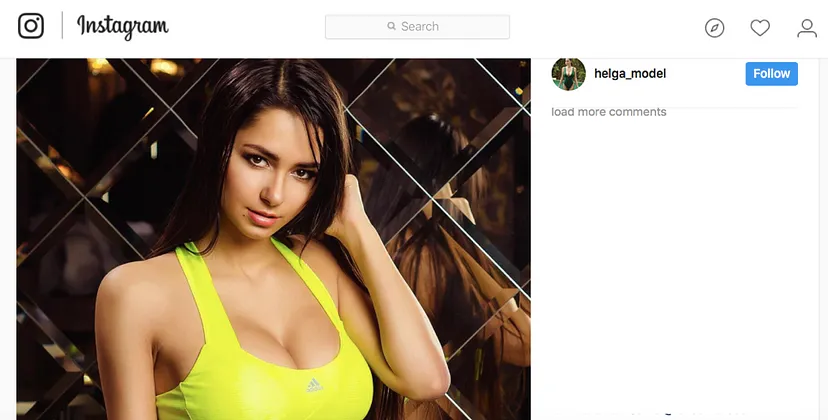
As @DFRLab has reported, using the image of a model to create a fake account is classic bot behavior. According to a reverse search of the image, it has been used by a number of other accounts with both male and female handles: @LindaDo31357375, @AnnEste4131598, @FLillianAshley20, @RalphRandall12, @LewisHaney4. As of June 29, all of these accounts were suspended.
The “Destiny Shelton” account is thus both an anonymous account and an amplifier, two of the three identifying characteristics of a probable bot. Its activity rate is an average of 49.7 engagements (tweets and likes) per day, fractionally under the rate of 50 tweets a day identified by Oxford University’s Computational Propaganda Project as suspicious.
The likelihood is that this, at least, is a bot.
Another account, @engelderliebe80, posted ten retweets of #AfDLeaks without any original posts. The account gives no personal information, and as of June 28, all of its 100 most recent posts were retweets. In the 30 days prior to that date, it had posted 5,586 tweets, at an average rate of 186 per day. This is bot-like behavior.
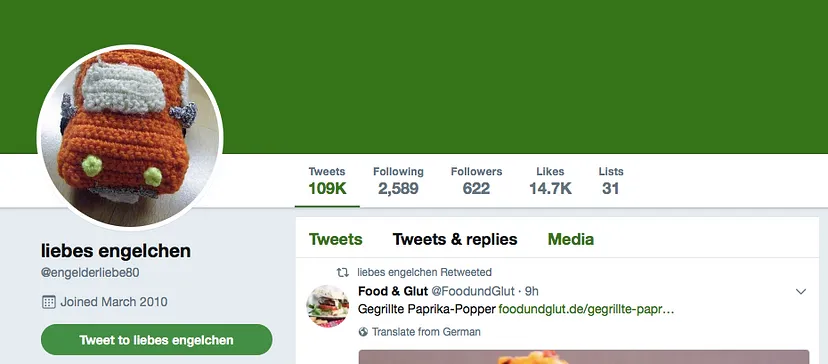
However, the majority of accounts that tweeted on #AfDLeaks showed some human characteristics, posting a significant proportion of original tweets and replies, even if their activity levels were very high.
For example, the account @yoga_and_more retweeted ten#AfDLeaks posts, with no authored posts of its own — actions that indicate a pattern of amplification. It has posted over 151,000 tweets since its creation in July 2015, at an average rate of around 215 per day. The account gives no personal identification other than a screen name. These characteristics are typical of bots.
However, a significant number of its most recent posts as of June 28 were replies that appeared to be authored, and that contained a high number of emojis. This suggests that the account is at least partly human-operated:
Such replies could, in theory, be generated by a chatbot programmed to reply. If so, this is a more sophisticated bot than the simple amplifiers that tend to drive large Twitter campaigns.
It is unclear what effect accounts such as these had on overall traffic. One potential indicator is that the 50 most active accounts produced 579 tweets on #AfDLeaks over a week, making up 18% of total traffic.
By contrast, the 50 most active accounts that tweeted on #LaFranceVoteMarine posted 11,408 in a few hours, making up 24% of all traffic. The 50 most active accounts to mention the Kremlin propaganda outlet RT in a separate study in April posted 7,388 tweets, or 32% of all mentions.
Thus the most active accounts — including some whose behavior seems characteristic of automation — played less of a role in boosting traffic on #AfDLeaks than has been observed in other studies.
Conclusion
The low level of overall activity on #AfDLeaks, the gradual increase in traffic, the low average number of tweets per user, and the low proportion of tweets from the top fifty users all differ markedly from the traffic shown by hashtag drives such as #LaFranceVoteMarine.
Taken together, these indicators suggest that, despite the apparent presence of some automated or amplifier accounts, the traffic on #AfDLeaks was primarily organic and driven by interested users, rather than by a coordinated amplification network.
In terms of overall traffic, this significantly limited the hashtag’s impact. At their peak, #LaFranceVoteMarine and #MacronLeaks generated more tweets in a minute than #AfDLeaks did in an hour. However, #AfDLeaks had a longer effective lifespan, spread across two days.
Overall, the traffic on #AfDLeaks appears to be the result of organic interest, rather than artificial amplification. However, even in this environment, bot accounts appear to have played some role. The hashtag therefore serves as a case study of how hashtags spread organically; but it is also a reminder that Twitter bots are ubiquitous. Their presence is to be expected in all environments: what is important to analyze is whether they have a distorting effect on debate.