#BotSpot: Twelve Ways to Spot a Bot
Some tricks to identify fake Twitter accounts
#BotSpot: Twelve Ways to Spot a Bot
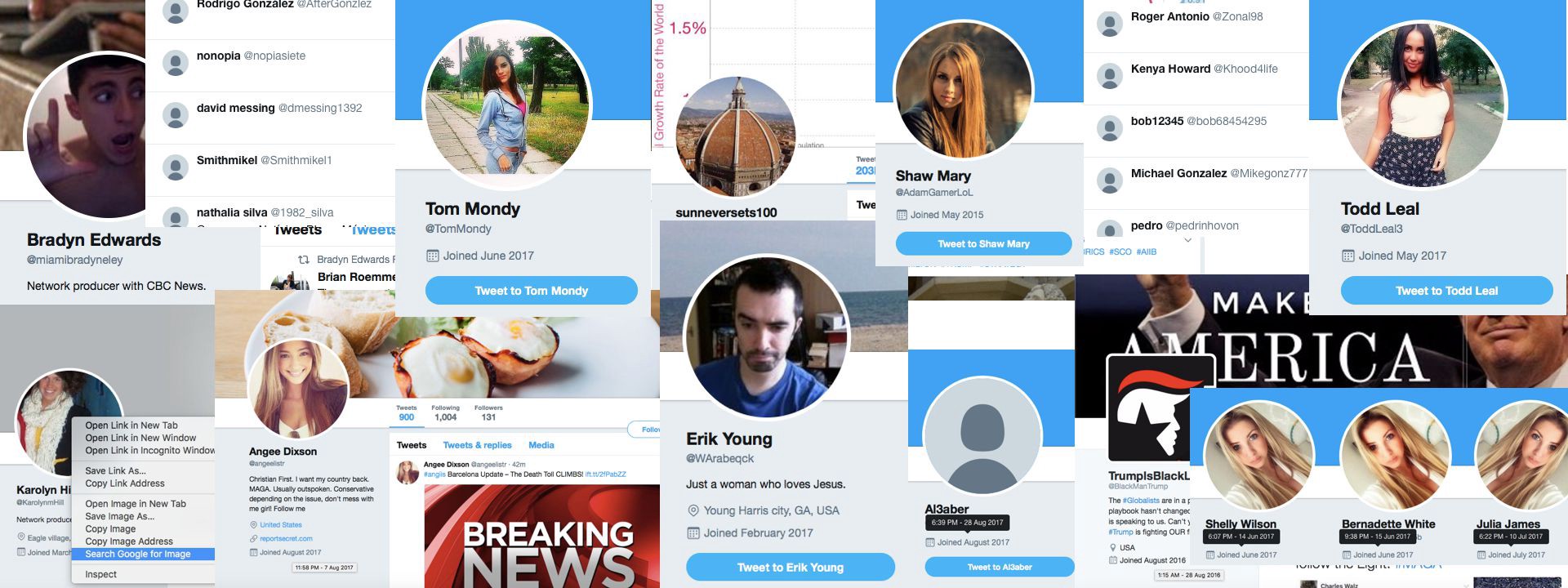
Some tricks to identify fake Twitter accounts

“Bots” — automated social media accounts which pose as real people — have a huge presence on platforms such as Twitter. They number in the millions; individual networks can number half a million linked accounts.
These bots can seriously distort debate, especially when they work together. They can be used to make a phrase or hashtag trend, as @DFRLab has illustrated here; they can be used to amplify or attack a message or article; they can be used to harass other users.
At the same time, many bots and botnets are relatively easy to spot by eyeball, without access to specialized software or commercial analytical tools. This article sets out a dozen of the clues, which we have found most useful in exposing fake accounts.
First principles
A Twitter bot is simply an account run by a piece of software, analogous to an airplane being flown on autopilot. As autopilot can be turned on and off, accounts can behave like bots and like human users at different times. The clues below should therefore be viewed as indicators of botlike behavior at a given time, rather than a black-or-white definition of whether an account “is” a bot.
His ancient bridge is thinking without the last saturated computer.
— PoetryBot (@AutoShakespeare) November 29, 2015
Not all bots are malicious or political. Automated accounts can post, for example, poetry, photography or news, without creating any distorting effect.
I can see still life photography, darkness, and musical instrument accessory. pic.twitter.com/M4uStMDwN1
— Cloud Vision Bot (@cloudvisionbot) August 28, 2017
Our focus is therefore on bots which masquerade as humans and amplify political messaging.
In all cases, it is important to note that no single factor can be relied upon to identify bot-like behavior. It is the combination of factors which is important. In our experience, the most signifcant three can be called the “Three A’s”: activity, anonymity, and amplification.
1. Activity
The most obvious indicator that an account is automated is its activity. This can readily be calculated by looking at its profile page and dividing the number of posts by the number of days it has been active. To find the exact date of creation, hover the mouse over the “Joined …” entry.

The benchmark for suspicious activity varies. The Oxford Internet Institute’s Computational Propaganda team views an average of more than 50 posts a day as suspicious; this is a widely recognized and applied benchmark, but may be on the low side.
@DFRLab views 72 tweets per day (one every ten minutes for twelve hours at a stretch) as suspicious, and over 144 tweets per day as highly suspicious.
For example, the account @sunneversets100, an amplifier of pro-Kremlin messaging, was created on November 14, 2016. On August 28, 2017, it was 288 days old. In that period, it posted 203,197 tweets (again, the exact figure can be found by hovering the mouse over the “Tweets” entry).
This translates to an average of 705 posts per day, or almost one per minute for twelve hours at a stretch, every day for nine months. This is not a human pattern of behavior.
2. Anonymity
A second key indicator is the degree of anonymity an account shows. In general, the less personal information it gives, the more likely it is to be a bot. @Sunneversets100, for example, has an image of the cathedral in Florence as its avatar picture, an incomplete population graph as its background, and an anonymous handle and screen name. The only unique feature is a link to a U.S.-based political action committee; this is nowhere near enough to provide an identification.
Another example is the account @BlackManTrump, another hyperactive account, which posted 89,944 tweets between August 28, 2016 and December 19, 2016 (see archive here), an average of 789 posts per day.

This account gives no personal information at all. The avatar and background are non-specific, the location is given as “USA” and the bio gives a generic political statement. There is thus no indication of what person lies behind the account.
3. Amplification
The third key indicator is amplification. One main role of bots is to boost the signal from other users by retweeting, liking or quoting them. The timeline of a typical bot will therefore consist of a procession of retweets and word-for-word quotes of news headlines, with few or no original posts.
The most effective way to establish this pattern is to machine-scan a large number of posts. However, a simpler, eyeball identification is possible by clicking on the account’s “Tweets and replies” bar and scrolling down the last 200 posts. The number 200 is largely arbitrary and is designed to give a reasonable and manageable, large sample; researchers who have more time and tougher eyeballs can view more.
As of August 28, for example, 195 of @Sunneversets100’s last 200 tweets were retweets, many of them from Kremlin outlets RT and Sputnik:

Showing one more degree of sophistication, most of @BlackManTrump’s posts until November 14 appeared to be retweets with the telltale phrase “RT @” removed:

Thus both @BlackManTrump and @Sunneversets show clear botlike behavior, combining very high activity, anonymity, and amplification.
As a caveat, it should be noted that @BlackManTrump was silent from November 14 to December 13, 2016; when it resumed posting, it was at a far lower rate, and with a higher proportion of apparently authored tweets. It would therefore be entirely correct to say that it behaved like a bot until mid-November, but not that it is a bot now.
Another amplification technique is to program a bot to share news stories direct from selected sites without any further comment. Direct shares are, of course, a standard part of Twitter traffic (readers are more than welcome to share this post, for example), and are not suspicious in themselves; however, an account which posts long strings of such shares is likely automated, as in this account opposed to U.S. President Donald Trump, identified in July:

4. Low posts / high results
The bots above achieve their effect by the massive amplification of content by a single account. Another way to achieve the same effect is to create a large number of accounts which retweet the same post once each: a botnet.
Such botnets can quickly be identified when they are used to amplify a single post, if the account which made the post is not normally active.
For example, on August 24, an account called @KirstenKellog_ (now suspended, but archived here) posted a tweet attacking U.S. media outlet ProPublica (propublica.com).

As the above image shows, this was a very low-activity account. It had only posted 12 times; 11 of them had already been deleted. It had 76 followers, and it was not following any accounts at all.
Nevetherless, its post was retweeted and liked over 23,000 times:

Similarly, the following day, another apparently Russian account posted an almost identical attack, and it scored over 12,000 retweets and likes:

This account is just as idle, having posted six tweets, the earliest on August 25, and it followed five other accounts:

It is beyond the bounds of plausibility that two such idle accounts should be able to generate so many retweets, even given the use of hashtags such as #FakeNews and #HateGroup. This disparity between their activity and their impact suggests that the accounts which amplified them belong to a botnet.
5. Common content
The probability that accounts belong to a single network can be confirmed by looking at their posts. If they all post the same content, or type of content, at the same time, they are probably programmed to do so.
In the suspected botnet which amplified @KirstenKellog_, for example, many of the accounts shared identical posts such as this:



Sometimes, bots share whole strings of posts in the same order. The three accounts below are part of the same anti-Trump network identifed in July:



On August 28, the same three accounts shared identical posts in identical order again; @ProletStrivings added a retweet to the mix:



Such identical series of posts are classic signs of automation.
6. The Secret Society of Silhouettes
The most primitive bots are especially easy to identify, because their creators have not bothered to upload an avatar image to them. Once called “eggs”, from the days when the screen image for an account without an avatar was an egg, they now resemble silhouettes.
Some users have silhouettes on their accounts for entirely innocuous reasons; thus the presence of a silhouette account on its own is not an indicator of botness. However, if the list of accounts which retweet or like a post looks like this…

… or if an account’s “Followers” page begins to look like the meeting place for the Secret Society of Silhouettes…

…it is a certain sign of bot activity.
7. Stolen or shared photo
Other bot makers are more meticulous, and try to mask their anonymity by taking photos from other sources. A good test of an account’s veracity is therefore to reverse search its avatar picture. Using Google Chrome, right-click on the image and select “Search Google for Image”.

Using other browsers, right-click the image, select “Copy Image Address”, enter the address in a Google search and click “Search by image”.


In either case, the search will show up pages with matching images, indicating whether the account is likely to have stolen its avatar:

In the case of “Shelly Wilson”, a number of accounts in the same network actually used the image, confirming that they were fakes:



8. Bot’s in a name ?
A further indicator of probable botness is the handle (account name starting with “@”) that it uses. Many bots have handles which are simply alphanumeric scrambles generated by an algorithm, such as these:

Others have handles which appear to give a name, but it does not match the screen name:



Yet others have typically male names but female images (an occurrence which appears far more common among bots than a female handle with a male image, perhaps to target male users)…



… or male handles, but female names and images…



… or something different entirely.

All these indicate that the account is a fake, impersonating someone (often a young woman) to attract viewers. Identifying the type of fake, and whether it is a bot, will depend on its behavior.
9. Twitter of Babel
Some bots are political, and only ever post from one point of view. Others, however, are commercial, and seem hired out to the highest bidder regardless of the content. Most of their posts are apolitical; but they, too, can be used to boost political tweets.
Such botnets are often marked by extreme diversity of language use. A look at the retweets posted by Erik Young, the “woman who loves Jesus,” for example, shows content in Arabic and English, Spanish, and French:



A similar look at posts from the anonymous and imageless account @multimauistvols (screen name “juli komm”) shows tweets in English…

… Spanish…

… Arabic…

… Swahili (according to Google Translate)…

… Indonesian…

… Chinese…

… Russian…

… and Japanese.

In real life, anyone who has mastered all those languages probably has better things to do than advertising YouTube videos.
10. Commercial content
Advertising, indeed, is a classic indicator of botnets. As noted above, some botnets appear to exist primarily for that purpose, only occasionally venturing into politics. When they do, their focus on advertising often betrays them.
A good example is the curious net of bots which retweeted a political post from an account, @every1bets, usually devoted to gambling.
The retweeters claimed a variety of identities, as this listing shows:

But they all tended to post a high proportion of commercials.



Accounts which largely show retweets like this, especially if they do so in multiple languages, are most probably members of commercial botnets, hired out to users who want to amplify or advertise their posts.
11. Automation software
Another clue to potential automation is the use of URL shorteners. These are primarily used to track traffic on a particular link, but the frequency with which they are used can be an indicator of automation.
For example, one recently-exposed fake account called “Angee Dixson”, which used the avatar image of German supermodel Lorena Rae, shared a large number of far-right political posts. Every one was marked with the URL shortener ift.tt:

This is a type of software produced by a company called ifttt.com, which allows users to automate their posts according to a number of criteria — for example, retweeting any post with a given hashtag. A timeline which is full of ift.tt shorteners is therefore likely to be a bot.
Other URL shorteners can also indicate automation, if they occur repeatedly throughout the timeline. The shortener ow.ly, for example, is attached to social media manager HootSuite; some bots have been known to post long strings of ow.ly shares from websites, indicating likely automation. Twitter’s own TweetDeck facility allows users to embed a variety of URL shorteners, such as bit.ly or tinyurl.com.
Yet again, the use of such shorteners is part and parcel of online life, but an account which obsessively shares news articles using the same shortener should be assessed for other indications that it is a bot.
12. Retweets and likes
A final indicator that a botnet is at work can be gathered by comparing the retweets and likes of a particular post. Some bots are programmed to both retweet and like the same tweet; in such cases, the number of retweets and likes will be almost identical, and the series of accounts which performed the retweets and likes may also match, as in this example here:


In this example, the variation between the number of retweets and likes is just 11 responses — a difference of less than 0.1 percent. Exactly the same accounts retweeted and liked the tweet, in the same order, and at the same time. Across a sample of 13,000 users, this is too unlikely to be a coincidence. It indicates the presence of a coordinated network, all programmed to like, and retweet, the same attack.
Conclusion
Bots are an inseparable part of life on Twitter. Many are entirely legitimate; those which are not legitimate tend to have key characteristics in common.
The most common indicators are activity, anonymity, and amplification, the “Three A’s” of bot identification; but other criteria also exist. The use of stolen images, alphanumeric handles, and mismatched names can reveal a fake account; so, too, can a slew of commercial posts, or posts in a wide range of languages.
What is most important, however, is awareness. Users who can identify bots themselves are less likely to be manipulated by them; they may even be able to report the botnets and have them shut down. Ultimately, bots exist to influence human users. The purpose of this article is to help human users spot the signs.
Follow along for more in-depth analysis from our #DigitalSherlocks.