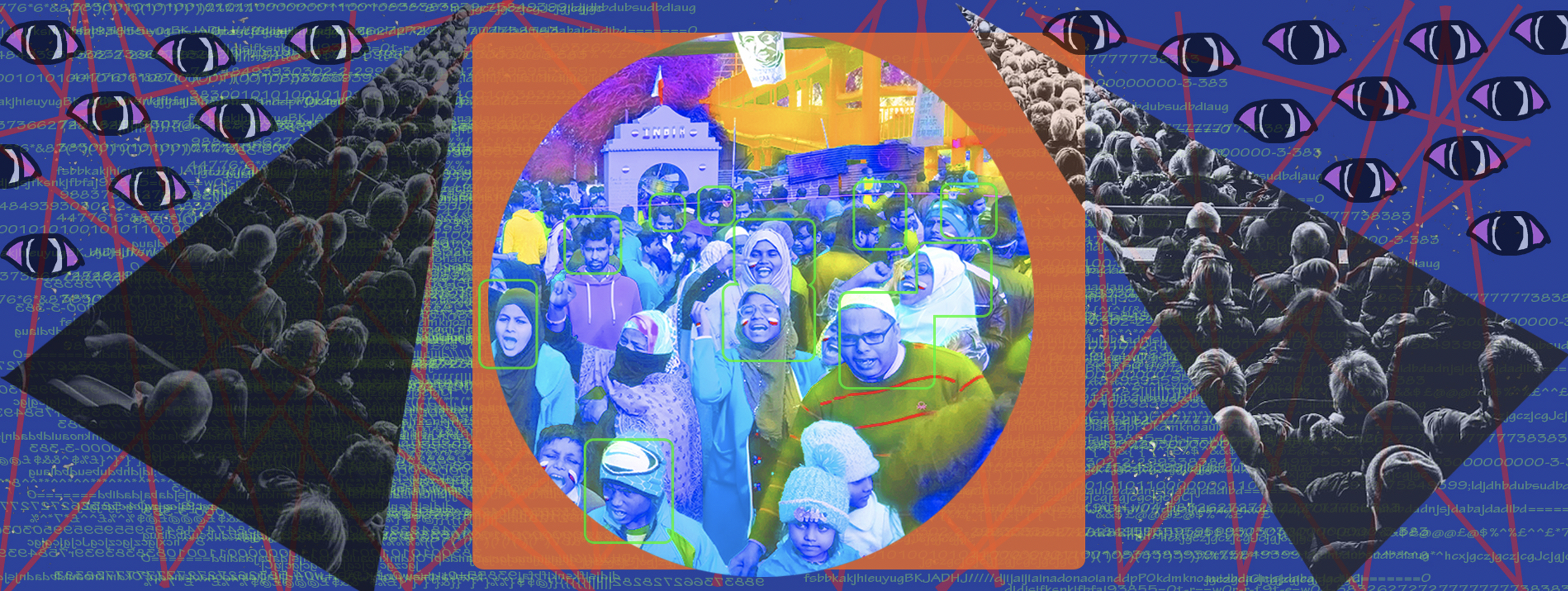
Facial recognition technology poses challenges to human rights and democratic accountability
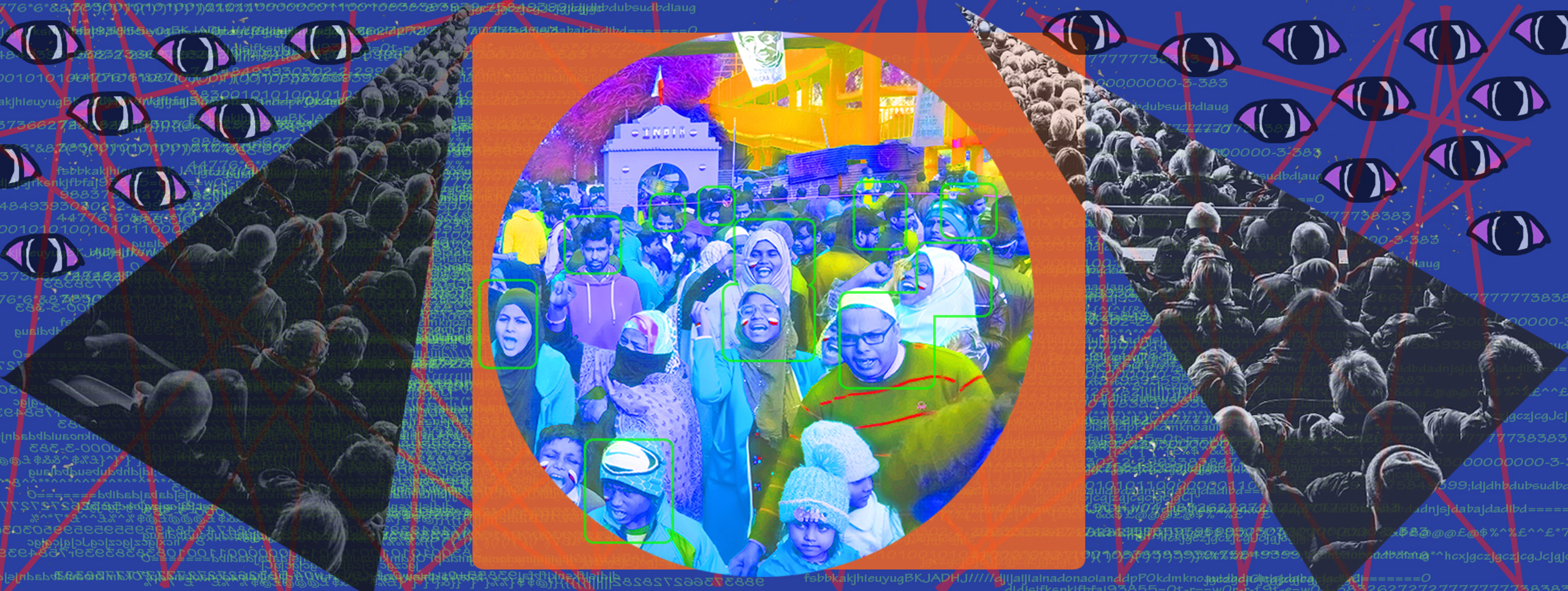
As part of our effort to broaden expertise and understanding of information ecosystems around the world, the DFRLab is publishing this external contribution. The views and assessments in this op-ed do not necessarily represent those of the DFRLab.
Artificial Intelligence technologies are often presented as evidence of society’s progress toward a utopian vision: a data-driven, corruption-free world that has eradicated individual greed and human error. Instead, the increasing adoption of such systems by a range of governments around the world indicates that these automated systems can also be emphatically anti-democratic, empowering mass surveillance with little accountability and oversight, and serving as a powerful tool for the harassment of dissenting voices.
In the wake of the recent nationwide Black Lives Matter protests that swept across the United States, multiple U.S.-based technology companies, including IBM, Amazon, and Microsoft, made efforts to scale back their involvement in technologies that enable police violence. Yet these same companies continue to sell and trade AI-based surveillance technologies in a number of countries, including India. Over the past five years, the world’s largest democracy has experienced the unprecedented adoption of facial recognition technology (FRT) in the absence of any oversight or safeguards.
In 2019, the Government of India called for bids to develop an Automated Facial Recognition System (AFRS), which would potentially be the world’s largest facial recognition system, building upon an integrated digital backend of various policing databases from across the country. FRT is already extensively deployed by various law enforcement agencies, however. As of the time of writing, at least 17 police departments in India have implemented or proposed the use of FRT. Most recently, these tools were used to target, profile, and surveil peaceful demonstrators against the Indian government’s divisive citizenship law, as well as to investigate individuals allegedly involved in the Delhi riots.
How effective is FRT?
Facial recognition technology refers to automated systems that collect and process data about an individual’s face. Privacy experts have expressed concern regarding the continued widespread adoption of such systems, warning that they can be highly intrusive. They rely on the capture, extraction, storage, and transfer of individual citizens’ biometric facial data, often without their express consent.
As a result, the use of such technologies in countries where law enforcement officers use AI, particularly systems like FRT, has become a subject of increased debate in recent years, with many privacy and human rights advocates highlighting how these forms of surveillance have been enabled by large technology companies located in the U.S., Europe, and China.
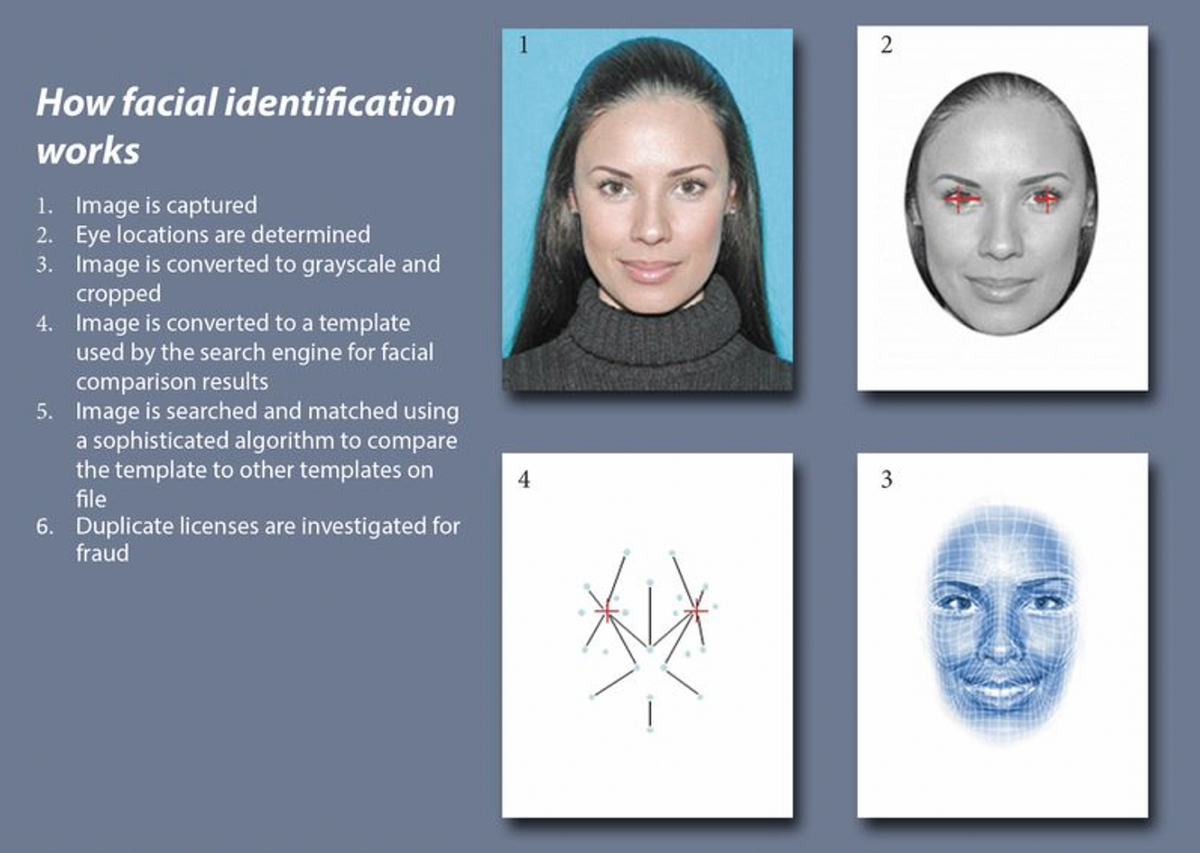
Due to the “black box” nature of FRT technologies, their performance must be audited or tested against so-called “benchmarks,” or databases assembled for auditing purposes, to define their accuracy. Accuracy, however, is a highly contingent term, considering that FRT essentially employs statistical modelling techniques, the performance of which is dependent upon the features of the samples from which the model is derived, as well as the samples to which it is applied. The actual performance of FRT, particularly on populations that are underrepresented in either training or benchmarking databases, can be significantly different from its accuracy as determined by the benchmarks.
While no systematic framework for audits of FRT currently exists in India, court and police records indicate that uses of FRT in India have been disastrously inaccurate. In March 2020, Indian Home Minister Amit Shah declared that Delhi Police had utilized FRT to identify more than 1,100 individuals allegedly involved in the outbreak of sectarian violence in the capital in February 2019. According to reporting by The Print, an Indian publication that investigated documents submitted to the Delhi High court by the enforcement authorities, the facial recognition system utilized by the Delhi police had initially been acquired to identify missing children. In 2018, the system operated at an accuracy rate of 2 percent before dropping to 1 percent in 2019. That same year, the Ministry of Women and Child Development revealed that the system could not even distinguish between boys and girls, raising concerns regarding the veracity of its assessments.
Even if one assumes FRT can be perfectly accurate across diverse populations, as a technology that relies on databases, the effect of FRT will be felt only on those who have been added to a database — for example, in criminal records. This, in turn, is a contested process that incorporates historical biases or discrimination. There is a danger that such biases may be reproduced at scale in India, particularly when considering that many of the countries criminal records databases continue a legacy of racist and casteist assumptions about “criminal tribes.”
Surveillance without borders
AI-based surveillance relies upon distributed assemblages of hardware, databases, algorithmic models, and institutional processes. Contemporary FRT utilizes machine-learning algorithmic models to extract the most relevant features of faces from an image for identification and compare them with a new database. The choice and weights of features to be extracted are “learned” through databases created for this purpose. FRT therefore relies extensively upon the availability of databases that can be purposed or repurposed for the training of machine-learning algorithms, which in turn influence the performance of the algorithm, based on facial characteristics captured in the underlying database.
Accordingly, a training database that is not representative of the target group being surveilled is likely to be inaccurate. An analysis of three commercially released facial-analysis programs by researchers from MIT and Stanford University found that the algorithms demonstrated gender and skin-type biases, exhibiting an error rate of 0.8 percent for light-skinned men while increasing to 34.7 percent when identifying dark-skinned women.
An analysis of the use of FRT systems by law enforcement authorities in India indicates how it is inextricable from the dynamics of global trade that makes up the modern AI industry. Transborder surveillance is implicated in almost every implementation of FRT in India. HikVision, a Chinese firm with close ties to the Chinese government, supplies the hardware for upwards of 100,000 CCTVs in New Delhi that collect and collate the video data for the FRT software. Corporations including Japan’s NEC and the United States’ Clearview provide the facial matching software to profile and identify individuals from the captured data.
While little is known about the training databases relied upon by FRT vendors in India, studies have indicated the increasing transborder use of databases — including Microsoft’s MSCeleb or the Megaface database — in law enforcement contexts by authoritarian regimes, such as the Chinese Communist Party’s repression of minority populations. In this manner, these massive databases, ostensibly released for encouraging “cutting-edge” research in AI, end up fuelling violence and incarceration through FRT.
Analyses of the global surveillance industry indicate that it is concentrated in the United States, Europe, and China, with companies in these countries providing services to a range of governments in South Asia and Africa that do not currently have effective privacy protections or legal accountability for the use of such invasive technologies. Accordingly, as the development of AI-technologies becomes increasingly embedded within transnational networks of trade and data, they become further distanced from the necessary conversations about responsibility, accountability and democratic governance that must be had with the populations in countries facing the brunt of surveillance, control and violence which these technologies enable.
Standard-setting institutions and bodies, a core component of the technology industries efforts at self-regulation, are also implicated in the transborder surveillance trade. A precondition for India’s proposed national FRT project is that the vendor must have been tested by the U.S. National Institute of Standards and Technology (NIST). A high score on the NIST test ostensibly provides a veneer of legitimacy for FRT developers. One Indian company supplying FRT to law enforcement has also claimed its technology has been benchmarked against the “Labeled Faces in the Wild” dataset, which was assembled by the University of Massachusetts Amherst with explicit disclaimers on its website that its benchmark is not indicative of an algorithm’s viability. Both the NIST and LFW benchmarks are known to indicate discrepant performances across different demographics. According to the latest forecast compiled by TechSci Research, an Indian market analysis firm, India’s domestic FRT industry is projected to grow from $700 million in 2018 to $4.3 billion by 2024.
Within this context, studies indicate that the intensification of surveillance of populations in countries like India benefits international corporations both through increased profitability without mandating any transparency or accountability on the behalf of the FRT system providers. This, in effect, shields these service providers from any culpability regarding the social and ethical challenges created by use of their products in a range of countries across the globe, particularly illiberal ones. In turn, the desire by governments in India and other countries in the region to institute such automated surveillance technologies has seen them sign over the privacy of nearly two billion citizens, as well as providing their countries as a testing ground for the continual experimentation and evolution of technologies within the field.
The story of FRT in India exemplifies the difficulties in governing increasingly transnational AI-based surveillance systems. Contending with these systems requires taking into account not only the companies engaging in technology transfers and trades, but also the ecosystem of norms and institutions guiding research and development into these technologies. This includes, for example, the availability and dissemination of “training data” consisting of (mostly non-consensually obtained) faces, as well as the institutional elements that exist to validate the use of such technologies, such as the benchmarking tests and standard setting institutions.
The techniques, practices, and institutions used to develop AI are intensifying surveillance and violence, particularly in countries with weak systems for preserving privacy or law enforcement accountability. The United Nations and Privacy International have pushed back against the global surveillance industry, calling for comprehensive export control regimes for technologies (such as the Wassenaar Arrangement, which prohibits exports of certain dual-use technologies), and advocating for stronger human rights safeguards in the countries of import, including moratoriums on technology export licenses. Despite this progress, a more coherent push to safeguard against the worst effects of the transborder surveillance trade is lacking.
While countries have leveraged trade restrictions in AI technologies to secure geopolitical gains, little has been done to place the development and trade in AI within a framework of privacy or human rights. The companies, governments, and institutions legitimizing and developing these technologies must have greater regard and accountability for their eventual manifestations as surveillance mechanisms outside of their direct control.
Ayushman Kaul is a Research Assistant, South Asia, with the Digital Forensic Research Lab.
Divij Joshi is an independent researcher and a Tech Policy Fellow with the Mozilla Foundation.