Making sense of foreign interference claims on the eve of the 2020 U.S. election
Introducing the DFRLab’s Foreign Interference Attribution
Making sense of foreign interference claims on the eve of the 2020 U.S. election
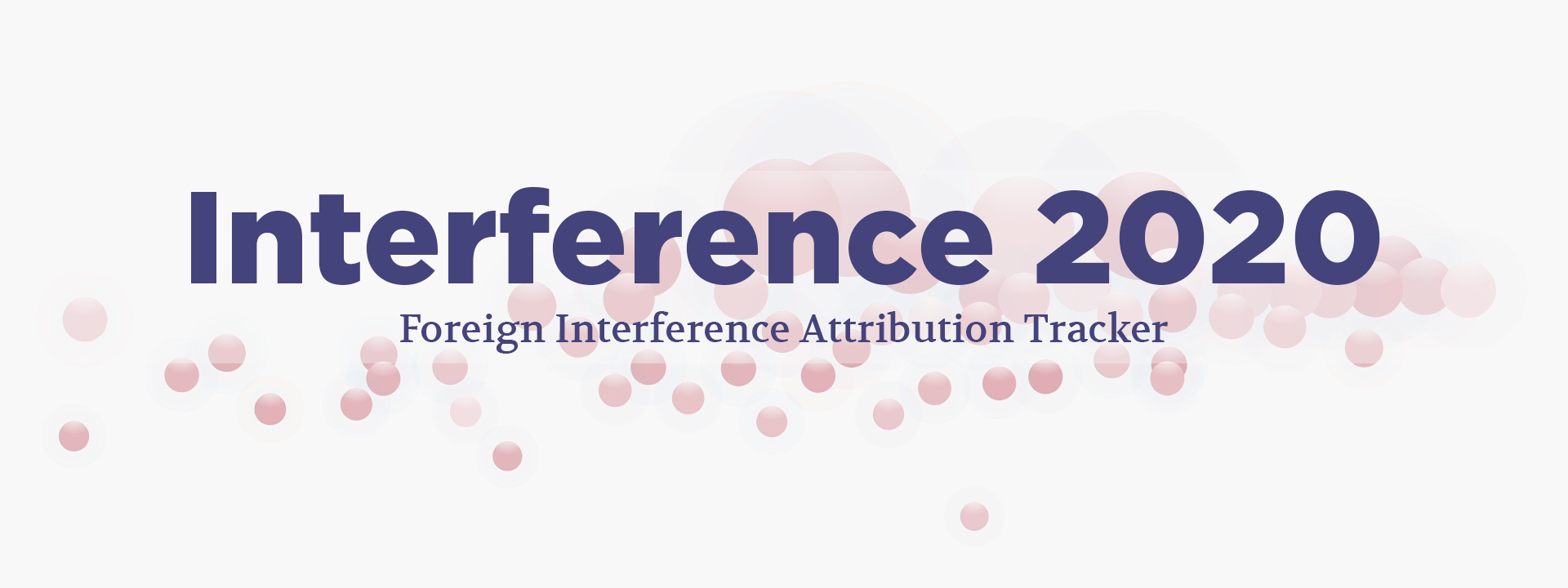
Introducing the DFRLab’s Foreign Interference Attribution Tracker
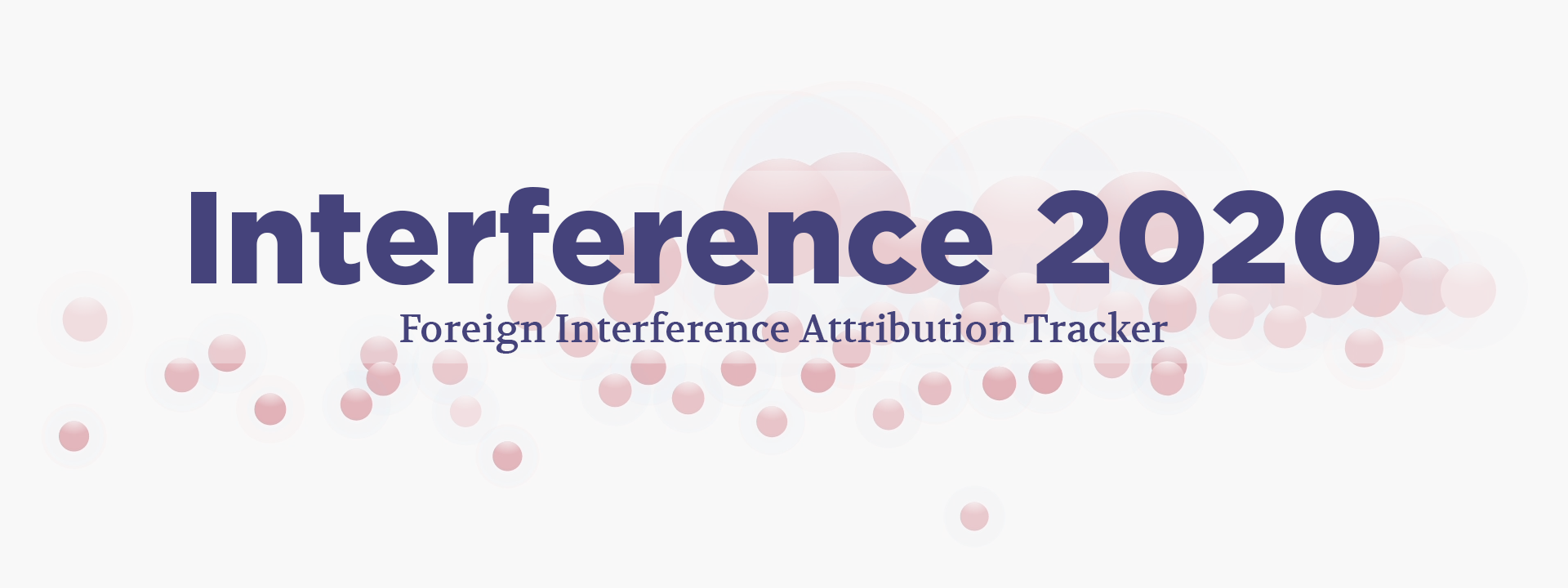
As the U.S. presidential election approaches, allegations of foreign interference have risen sharply. These claims have originated from U.S. intelligence agencies, technology companies, as well as political campaigns and senior political appointees serving in the current U.S. administration. According to DFRLab analysis, there have been at least 10 major foreign interference claims made in the month of September alone, each alleging different actors and different methods and objectives. These claims vary widely in their evidence and objectivity. Sometimes, they even contradict each other.
U.S. citizens are closely attuned to this issue. According to an August 2020 Pew survey, 75 percent of Americans believe that a foreign government will try to influence the 2020 election, with 62 percent of respondents describing it as a “major problem.” Even with many Americans paying attention, however, the volume of foreign interference claims has made it challenging for citizens to grasp the current reality of the problem. It has also made it more difficult for policymakers to respond to it, as well as for journalists to summarize and contextualize every instance or allegation of foreign interference adequately.
In order to address these concerns, the DFRLab is proud to launch its Foreign Interference Attribution Tracker (FIAT). This tool is an interactive, open-source database that captures allegations of foreign interference relevant to the 2020 election. It assesses the credibility, objectivity, evidence, transparency, and impact of each claim. FIAT currently tracks 65 cases involving 17 foreign nations. Both the tool and accompanying documentation can be accessed at interference2020.org.
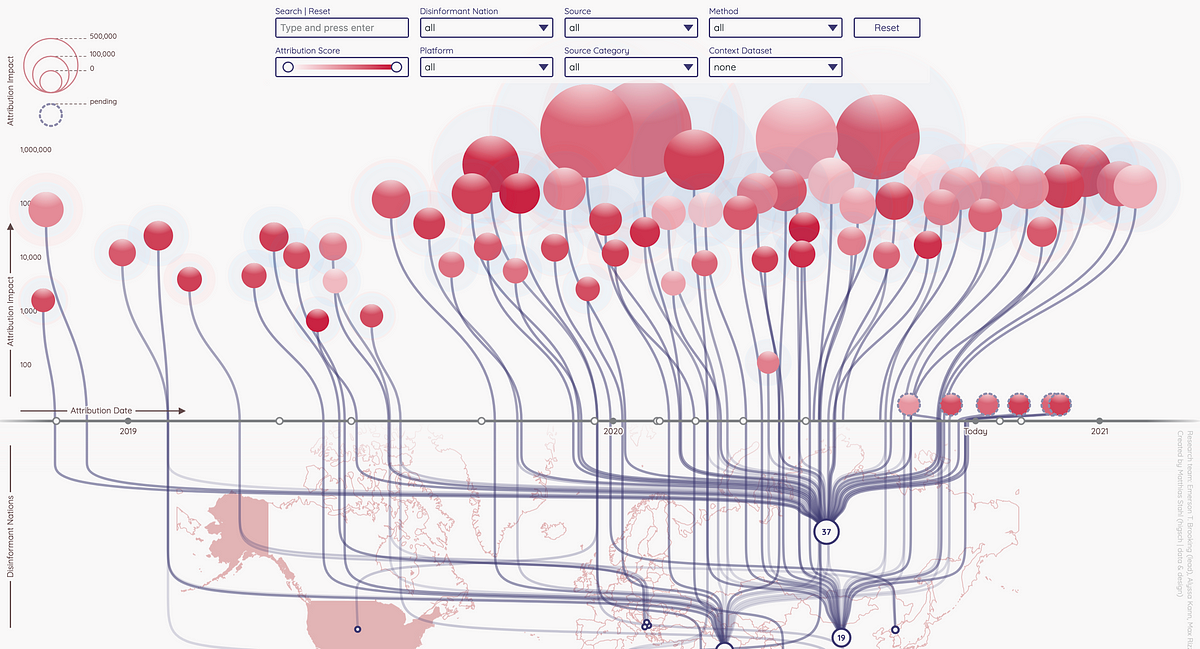
This project builds public attribution standards, provides an independent and reliable record of foreign interference in the 2020 election, serves as a resource for stakeholders about the evolving threat, and helps to build public resilience against future foreign interference efforts and disinformation. It has been created in service of the DFRLab’s mission to identify, expose, and explain disinformation and to promote objective fact as the basis for democratic governance worldwide.
FIAT will be regularly updated as further allegations of foreign interference in the 2020 election are made public. In the weeks to come, the DFRLab will add further functionality to the tool in order to build an enduring resource for both the disinformation research community and the general public.
How cases are selected
In order to be included, cases must fulfill three criteria:
First, cases must involve allegations of foreign interference by primarily digital means. The Australian Government Department of Home Affairs defines foreign interference as activity by a foreign actor that is “coercive, corrupting, deceptive, or clandestine” in nature, distinguishing it from the more benign phenomenon of foreign influence. By focusing expressly on digital activity, this definition denotes a range of interference activities — including disinformation, media manipulation, and cyber intrusion — that are conducted by foreign actors to affect political outcomes.
Second, cases must be novel. A novel case involves either a unique and newly arisen foreign interference claim or reveals new evidence to reinvigorate an old one. A novel case is also one in which significant newsworthiness is attached to the individual or organization making the claim. In general, a president or ex-president’s claim is novel regardless of the evidence presented. Meanwhile, an op-ed or report by a mid-level U.S. official is only novel if it contains previously undisclosed information.
Third, cases must be relevant to the 2020 U.S. election. This focuses case selection on alleged foreign interference that seems intended to influence voting behaviors, denigrate particular candidates, or engage in political or social issues of direct relevance to the election. It also bounds case selection to foreign interference claims that have occurred around or following the 2018 U.S. midterm elections. Several cases of clandestine, globally focused online networks with tangential interest in U.S. elections (such as operations attributed to Iran and Saudi Arabia) are included under this criteria, as they have still sought to exert some influence over U.S. election-related discourse.
Methodology
In order to assess and contextualize foreign interference claims properly, FIAT introduces two unique measurements: Attribution Impact and Attribution Score.
Attribution Impact measures the spread of case-related articles and content over the seven days following a foreign interference allegation. It is a sum of the cumulative Facebook engagements, Twitter shares, and Reddit engagements of relevant articles. The purpose of the Attribution Impact is to capture, in approximate terms, how much an attribution affected the U.S. news cycle. It is possible for highly credible, well-sourced claims to have very little media impact. Conversely, it is possible for salacious, poorly substantiated claims to dominate the news cycle.
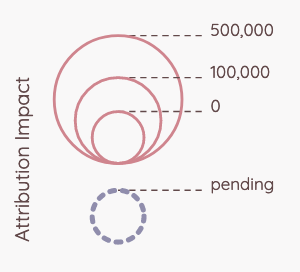
Attribution Impact is computed by a standardized query process that utilizes SerpApi (which enables the use of advanced Google search operators), BuzzSumo (which enables the query of a large database of social media content and collection of engagement data), and CrowdTangle (which enables the collection of engagement data for content not indexed by BuzzSumo). A detailed discussion of this process can be found in the “Methodology” section of interference2020.org.
Attribution Score, meanwhile, is a framework of 18 binary statements (true or false) intended to assess foreign interference claims made by governments, technology companies, the media, and civil society organizations. If a statement is deemed applicable, a point is awarded. If a statement is deemed inapplicable or irrelevant, no point is awarded. This scoring system is based on the experience of DFRLab experts in assessing — and making — such allegations. It is also based on a review of work produced by the wider disinformation studies community, and particularly resources compiled by attribution.news.

The Attribution Score is composed of four subsections:
Credibility
· The source of the attribution does not have a direct financial interest in a certain attribution outcome.
· The source of the attribution has a diversified and transparent funding stream.
· The source of the attribution does not strongly endorse a specific political ideology.
· The source of the attribution is in no way affiliated with a political campaign.
· The source of the attribution has not previously promoted mis- or disinformation.
Objectivity
· The attribution avoids using biased wording. The attribution avoids high-inference or emotive language.
· The headline accurately conveys the content of the attribution.
· The attribution clearly distinguishes factual information from argumentative analysis.
Evidence
· The attribution provides a clear illustration of the methods, tactics, and platforms involved in the alleged information operation.
· The attribution contextualizes the engagement with, and impact of, the alleged information operation.
· The attribution identifies actors and states allegedly responsible.
· The attribution clearly explains the strategic goal and rationale of the actors who conducted the alleged information operation.
· The attribution relies on information that is unique to, or can only be procured by, the relevant actor — e.g., classified information for U.S. federal agencies, back-end/developer information for technology companies.
Transparency
· The attribution provides open access to a dataset or archived links of alleged assets.
· The attribution methodology is clearly explained.
· The attribution is replicable through open-source evidence.
· The attribution acknowledges relevant limitations or mitigating factors in its assessment.
· The attribution has been corroborated by a third party or independent investigation.
An attribution that scores 15 points or higher is especially reliable. An attribution that scores six points or fewer should be carefully scrutinized.
Initial Findings
By sorting, evaluating, and visualizing foreign interference allegations, FIAT enables new insights into the timing and impact of these claims. Some initial observations:
· Two of the cases that had the most impact on the U.S. media environment are two of the least transparent in the dataset. These include vague news on February 21 that the Bernie Sanders campaign was made aware of Russian interference attempts by U.S. intelligence officials, as well as allegations on May 31 by unnamed U.S. officials of “foreign adversaries” infiltrating the George Floyd protests.
· The most transparent, evidence-driven foreign interference allegations have generally originated with U.S. technology companies. By contrast, allegations by U.S. agencies and officials have been generally unsupported by public evidence or documentation.
· The U.S. government is responsible for a disproportionate number of foreign interference allegations against China. Since the 2018 election, 37 foreign interference claims have been made against Russia and 19 against China. Among U.S. government officials, however, 14 claims have been made against Russia and 10 against China.
· All foreign interference allegations against China have occurred following the start of the COVID-19 pandemic in the United States. This is in stark contrast to allegations against Russia and Iran, which are more evenly distributed through the dataset.
In the weeks to come, the DFRLab will share more findings from the FIAT dataset. Readers can evaluate the data themselves by visiting interference2020.org.
The core FIAT research team is composed of Emerson T. Brooking, Alyssa Kann, Max Rizzuto, Jacqueline Malaret, and Helen Simpson. The tool was designed by Matthias Stahl. This project is directed by Graham Brookie and Emerson T. Brooking.