#ElectionWatch: Midterms Social Media Highlights American Divisions
Assessing partisan posts on Twitter ahead of the midterm elections in the United States
#ElectionWatch: Midterms Social Media Highlights American Divisions
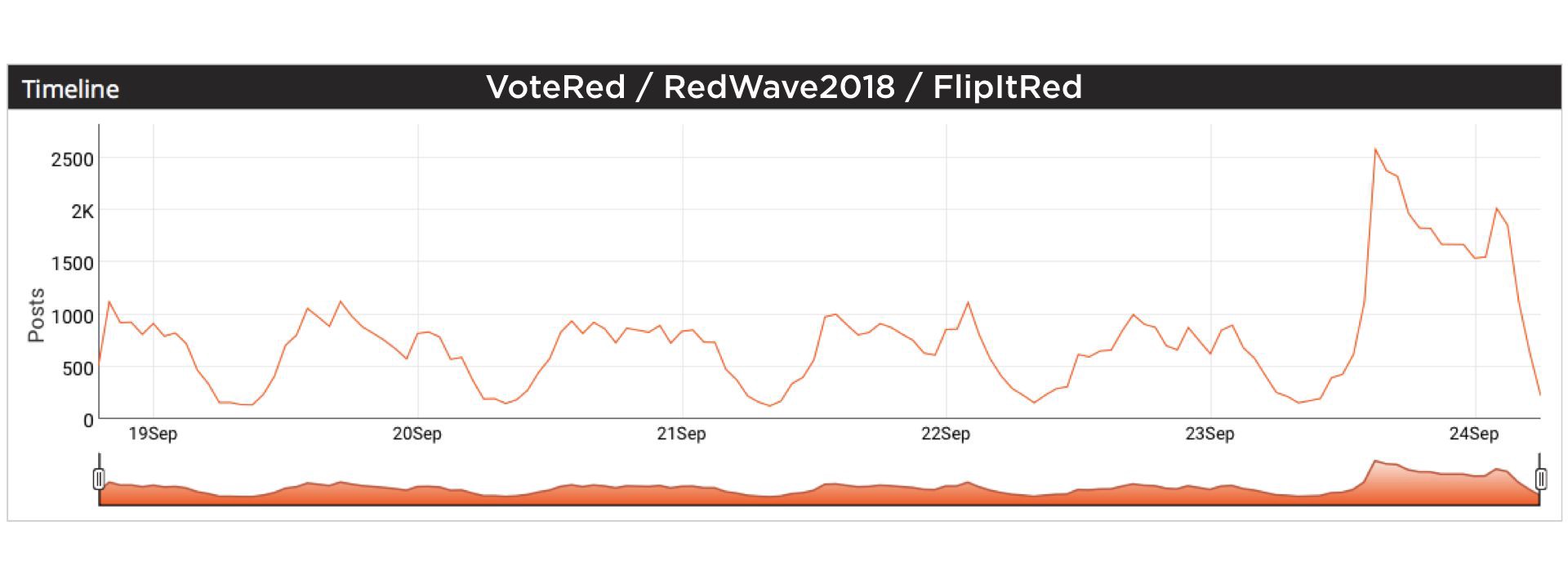
Assessing partisan posts on Twitter ahead of the midterm elections in the United States

Polarizing and hyper-partisan content is dominating the Twitter conversation in the United States around the midterm elections of November 6, according to an analysis of posts made in late September.
Supporters of the U.S. Democratic party were able to generate a greater overall volume of posts across two comparative scans of directly election-related hashtags, but supporters of the “alt-right” movement generated the highest engagement on an individual post. On both sides, polarized descriptions of the opposing party drove traffic.
The snapshot illustrates a deeply divided U.S. electorate, and an online environment which continues to reward polarizing rhetoric. These factors are likely to worsen after the elections.
“Blue” Series: More Posts, More Users
@DFRLab accessed Twitter’s public application programming interface (API) to conduct paired machine scans of three partisan slogans from each side of the debate: the Democratic “VoteBlue,” “BlueWave2018,” and “FlipItBlue,” and the Republican “VoteRed,” “RedWave2018,” and “FlipItRed.” These were composite “OR” scans, with all three slogans in a single search.
The hashtags were chosen for their direct reference to the election, and the ability they offered to compare them directly. Other partisan hashtags, such as #MAGA and #Resist, generated far more traffic, but were used in many contexts outside the election itself. The scans did not distinguish between official party posts, supportive posts, and hostile posts.
The scans constitute a snapshot of a single period in a long campaign. As such, they illustrate directly election-related sentiment in the scan period, but cannot be taken as an indicator of voting intentions, or overall momentum.
The first pair of scans studied all posts (tweets and retweets) made over the 48-hour period of September 18-20, 2018. The “blue,” pro-Democratic scan returned 79,424 posts in total. The “red,” pro-Republican scan returned 46,799 posts in total, or 59 percent of the pro-Democratic volume.

The difference in volume was not caused by users of the pro-Democratic hashtags posting more often. According to the machine scan, 39,758 users posted on pro-Democratic slogans, while just 20,715 posted on pro-Republican ones.

While some latitude must be given for hostile uses of the key phrases (for example, Democratic users posting hostile comments about Republican hashtags, or vice versa), this wide disparity suggests that, over the 48 hours, significantly more pro-Democratic users engaged on directly election-related hashtags than did their pro-Republican counterparts.
“Red” Series: More Posts Per User
The second pair of scans studied the 100,000 most recent tweets up to September 24, 2018.
In the “blue” (Democratic) series, the 100,000 posts were generated by 41,633 users. In the “red” (Republican) series, the 100,000 posts were generated by 38,942 users.

These figures were far closer than in the time-limited scan. On this occasion, the size difference between the two communities was relatively small — 2,691 users, or a variation of roughly 7 percent.
The figures showed that users of pro-Republican hashtags were, on average, somewhat more active than their opponents. On average, each pro-Democratic user posted 2.40 times during the scan, while each pro-Republican user posted 2.57 times. By contrast, users of the pro-Democratic hashtags were somewhat more numerous that their rivals, though far less than in the earlier scan.
Tweets and Retweets
A third indicator of user engagement was the proportion of total traffic made up by raw retweets — that is, retweets without added comment — compared with the proportion of traffic made up of apparently authored posts. In general, the lower the percentage of raw retweets, the higher the user activity.
Both “blue” scans returned retweet levels of just below 80 percent. The “red” scans returned even higher levels, over 85 percent. These are high figures, unusual for purely organic traffic.
In each scan, the absolute number of apparently authored posts on pro-Democratic hashtags was roughly double the number of apparently authored posts on pro-Republican hashtags.

Such high proportions of retweets could be driven by bots, but could equally be driven by human users retweeting one another as part of follow-back arrangements. More analysis would be needed to confirm bot activity.
Taken together, these scans indicated that, during the study period, more users posted a proportionately higher volume of original content on the pro-Democratic hashtags. This suggests a broad and engaged user base, generating more overall traffic (significantly more, in the 48-hour scan) than their counterparts on directly election-related slogans.
Alt-Right Drivers
While pro-Democratic hashtags dominated overall, the single most effective post in the scanned period, measured in the number of retweets it generated, was on a pro-Republican hashtag.
According to our machine scan of 100,000 posts, the pro-Democratic traffic followed a regular pattern, with roughly the same level of engagement each day, peaking at 1,500–2,000 posts per hour. The pro-Republican traffic largely followed the same pattern, peaking at around 1,100 posts per hour.
However, on September 23, the pro-Republican traffic surged to a peak of just over 2,500 posts an hour — almost double the usual rate of engagement, and the highest rate of any traffic in our scans.

This abrupt spike in traffic was driven by a single post from alt-right commentator Dinesh D’Souza, who counts just over one million Twitter followers. The post garnered over 13,000 retweets in the course of the scan, and over 15,000 by September 26.

This was the most-retweeted post on the pro-Republican hashtags by almost an order of magnitude. Retweets of the post accounted for one in every eight tweets in the scan of 100,000 posts.

It was also retweeted three times more often than the most-retweeted pro-Democratic post, which numbered just under 4,000 retweets during the equivalent scan.

This indicates the significant power that individual alt-right commentators have to generate very high levels of engagement, well above the usual flow of directly election-related traffic.
Polarized Posts
D’Souza’s post was the best-performing in any scan, measured by the number of retweets, but it was not the only aggressively partisan post. The best-performing tweet in the scan of 100,000 “blue” posts also characterized the opposing party in polarizing terms, accusing the Republican party as whole of “great malice” towards women, based on the party’s support for conservative Supreme Court nominee Brett Kavanaugh.

The best-performing pro-Democratic tweets in the timed scan also presented a black-and-white vision of the Republican party’s attitude towards women. It was retweeted over 11,000 times in the scan, and another 4,000 times thereafter, ultimately just overtaking D’Souza’s post.

Only one scan, the “red” time-limited one, returned a positive post as the top message.

As well as being the most positive, this was the least retweeted of the top tweets in the four scans, suggesting that the online communities on both sides of the divide gave greater weight to negative messaging about their opponents during the scanned period.
Automated Behavior
The overall pattern of traffic was consistent with organic activity. On both sides of the divide, however, individual users showed behavior patterns more characteristic of automated accounts than humans. This was particularly so among users of pro-Democratic hashtags.
According to the 48-hour scans, eight users posted more than 100 tweets in the scan period; of these, seven posted on pro-Democratic phrases.

One user stood out: @jgionova, which posted 581 times on pro-Democratic phrases in the 48-hour period, or an average of 12 times per hour. This account’s behavior was bot-like. Its profile, which gave no verifiable personal information, stated that it was created on July 12, 2016; by September 24, 2018, it had posted 269,589 times, for an average of 607.2 posts per day.

A Twitter search for authored posts from the account, as opposed to retweets, returned no posts more recent than April 13, 2018.

A separate scan of the account’s activity over the week of September 18–25, using the Twitonomy online tool, showed that it had posted 3,195 times, every one of them a retweet.

The Twitonomy scan further revealed that all but one of those retweets had been posted by an app called “JGio Bot.” Clicking on the link led to a request for permission to access Google from a website called labnol.org, which offered an online service to make Twitter bots.

The labnol.org page underlined that Twitter has rules against automated spamming; the “JGio bot” evidently ignored its strictures.
Follow-Back Teams
Other pro-Democratic accounts were highly active, and showed some characteristics of bot-like behavior, but interspersed these with authored posts, suggesting that they were either semi-automated cyborgs, or operated by highly-active human users.
The account @rjoseph7777, for example, posted 161 times in the 48-hour period, using over 2,000 hashtags in the process. Many of the posts consisted of headlines copied from anti-Trump news stories, followed by a string of pro-Democratic or anti-Trump hashtags, including #FBR, short for “Follow Back Resistance.” This is bot-like behavior.

However, among its posts were authored tweets and replies, including replies and comments on the news it was sharing, revealing it as at least partially human-operated.

One noteworthy feature of this account was the number of accounts it was following, in line with the “Follow Back Resistance” hashtag, #FBR. As of September 26, 2018, it was following 21,400 accounts, and followed by 22,700 accounts. Such a very high number of followed accounts, and the similarity of the number of followers and followed, supports the conclusion that this account was part of an organized follow-back community, whose purpose was to boost the American left’s footprint online.

Such communities certainly exist on the pro-Democratic side. A Google search for the hashtag #FBR led to a page called “Twitter Follow Back Resistance #FBR #FBResist,” which urged users to follow members of its list and follow them, in return for reciprocal follows.

Other highly-active accounts posting on the pro-Democratic hashtags also followed thousands of other users; in some cases, the numbers of followers and followed were almost identical, suggesting a follow-back arrangement.



These follow-back arrangements are common among political groups on Twitter. They are of interest because they reveal a level of community organization, and a determination to maximize the group’s impact online.
Highly-active accounts on the pro-Republican hashtags also showed clear signs of such community organization, including follow-back arrangements. Some also exhibited botlike behavior, though on a significantly lower level of activity than the pro-Democratic @JGionova.
For example, @Golfman072, which posted 78 times on the “red” series during the 48-hour scan, followed over 44,000 accounts, and was followed by over 40,000.

It repeatedly posted the same string of hashtags at intervals of less than a minute, suggesting that the account was either automated to repeat the same content, or managed by a human user copying and pasting content into a series of posts.

Other posts by the same account featured strings of user handles, in the same order, and with the same handles misspelled, shown by their appearance in black rather than blue.

Some of the most active posters of the pro-Republican hashtags followed tens of thousands of others, often almost identical with the number of followers, suggesting follow-back arrangements.



Again, this pattern of follow-back arrangements was overt, and actively promoted by some of the accounts, with instructions on how to maximize the community.

This self-organization within groups was common to both sides. It should not be taken as malicious behavior; it shows the level of engagement by partisans on both sides of the divide, and the strong similarity between their tactics.
Conclusions
Overall traffic patterns in this specific case study showed that pro-Democratic hashtags on directly election-related hashtags outperformed the equivalent pro-Republican ones. The “blue” series generated more tweets over a set time period; came from more users over a set number of posts; and included a higher volume of authored posts.
The “blue” series was materially boosted by one demonstrable bot. Other highly-active accounts on both sides of the partisan divide appeared partially automated, posting a high proportion of potential automatically generated content, but with an admixture of authored content.
Users on both sides benefited substantially from follow-back arrangements, aimed at maximizing their online impact. The techniques on both sides were similar, and produced similar results, in terms of the large numbers of followers such accounts had.
On both sides, polarized, negative messaging generated substantially more engagement than positive messaging. D’Souza’s crude characterization of the “good guys” and “evil party,” and @ProudResister’s post on the Republican attitude to women, both scored over 15,000 retweets, far more than the best-performing positive message.
Taken together, these factors reveal an online discussion which is already polarized, and is likely to become more so. The snapshot scans indicated that the overall momentum on directly election-related hashtags, measured in the number of posts and users, was with pro-Democratic users during the scanned periods, but that alt-right supporters still had the ability to generate very substantial traffic. Whichever side claims victory in the midterms, the other can be expected to respond with still more hostility. Given the emphasis given to negative messaging by both sides, America’s online polarization is likely to increase.
Follow along for more in-depth analysis from our #DigitalSherlocks.