#ElectionWatch: Bots on Both Sides in India
Automated accounts drive pro- and anti-Modi traffic
#ElectionWatch: Bots on Both Sides in India
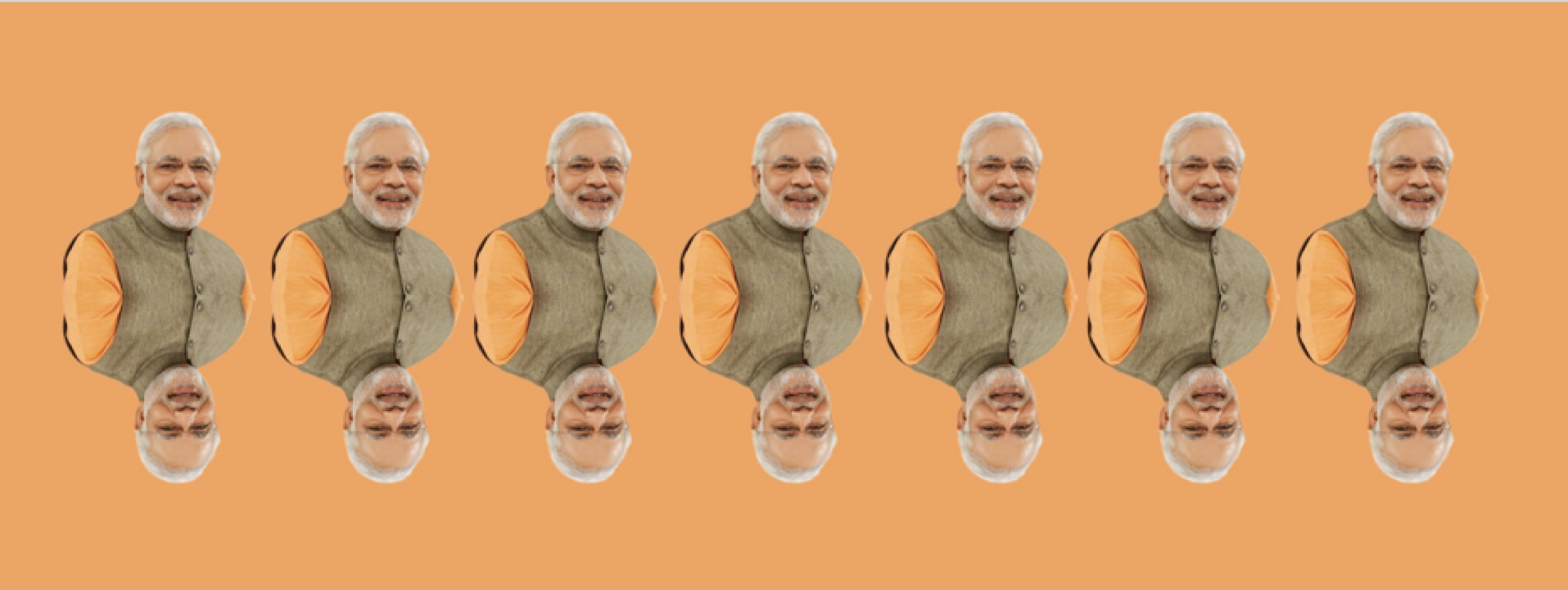
Automated accounts drive pro- and anti-Modi traffic

Automated Twitter “bot” accounts made a massive attempt to boost traffic on the platform in India in February, as the countdown to the world’s largest general election began.
The accounts were deployed on a massive scale on February 9-10 and boosted hashtags both in support of and in opposition to incumbent Prime Minster Narendra Modi, with small groups of accounts pushing out thousands of posts an hour. The accounts were domestic in origin and substance.
The incident highlights the sheer scale of attempts to manipulate Twitter traffic as India’s main political parties head to the polls. It also underlines the extent to which social media more broadly has become an electoral battleground.
India’s 875 million voters will be heading to the polls between April 11 and May 18. The ruling Bharatiya Janata Party (BJP), led by Modi and party president Amit Shah, while still the favorite, has recently slipped in the polls. The major political parties have launched large-scale electoral campaigns with a significantly larger focus on digital strategy, compared to the previous election in 2014.
While bots were used on both sides on February 9–10, the pro-Modi traffic was far more heavily manipulated than the anti-Modi traffic and, indeed, far more heavily manipulated than any large-scale traffic flow the DFRLab has analyzed as of yet. Conversely, while the scale of the activity was vast, its impact was rather muted given the relatively low number of followers of the accounts. The massive scale of the attempted manipulation nevertheless bodes ill for the quality of online debate in India as the election approaches.
Twitter’s systems are designed to detect large-scale automated efforts like this without necessarily suspending the accounts straight away: the bot traffic is therefore remarkable for the sheer effort it represents, rather than for its impact.
It remains important to be able to expose such efforts. The appendix to this article sets out one method for doing so, for the benefit of the open-source community, and identifies the record-breaking rate at which the accounts were posting.
#TNwelcomesModi
The DFRLab scanned traffic on the hashtag #TNwelcomesModi, short for “Tamil Nadu welcomes Modi,” which trended in India on February 9–10 and was mentioned over 777,000 times in two days. The hashtag referenced Modi’s visit to the southern state, where the BJP is historically weak.

The DFRLab analyzed the first 49,727 tweets in the flow to see whether the hashtag started to trend because of widespread interest or because it was pushed by a small group. The number was as close to 50,000 as could be retrieved by the scan, which was limited to a maximum of 50,000 for technical reasons. The scan covered 7 hours and 48 minutes of traffic.
The analysis used the Coefficient of Traffic Manipulation (CTM) method, which allows researchers to compare a given Twitter flow with known organic traffic, and traffic that was heavily gamed by small groups. The CTM method is described at the end of this article. The method was first published by the Oxford Internet Institute’s Computational Propaganda project.
The CTM assigns each traffic flow a score, based on how much it appears to have been gamed. In earlier studies, organic, non-manipulated traffic typically scored a CTM of 12 or lower. Heavily manipulated traffic that was boosted by bots and coordinated human users scored up to 60.

The first 49,727 tweets in #TNwelcomesModi scored a CTM of 123.98, the highest the DFRLab has ever recorded, indicating that it was very heavily manipulated by a very small group.
An “eyeball test” of the accounts that posted the hashtag most often confirmed that these accounts were bots. They have since been suspended. The most frequently posting account was @SasiMaha6, which posted #TNwelcomesModi tweets 1,803 times during the scan, or roughly one tweet every 15 seconds.

Another high-volume account was @priyamanaval6, which posted the hashtag 1,677 times, or roughly one tweet every 17 seconds for over seven hours.

Another high-volume account was @ANGEL0310970276, which posted 1,350 times, or roughly once every 22 seconds.

These sustained rates are far too high for human posting. The top three accounts alone posted #TNwelcomesModi 4,914 times, or roughly 10 percent of all traffic in the scan. The 50 most active accounts generated 30,446 tweets, or 61.2 percent of all traffic.
In other words, almost two-thirds of the posts that initiated #TNwelcomesModi and pushed it to trend came from just 50 accounts. This was an attempt at manipulation on an industrial scale, using a small number of hyper-tweeting bots to give the hashtag a massive boost.
#GoBackModi
The bot-driven praise for Modi’s visit was countered, in part, by bots. On February 10, the hashtag #GoBackModi also trended, pushing messages that supported the BJP’s main rival, the Congress Party.
This hashtag trended even faster, racking up 49,538 tweets in just over three hours in the early morning of February 10. It peaked at a lower rate, however, generating 447,000 posts on February 9–10.

The DFRLab analyzed 49,538 posts from the period when the hashtag accelerated most rapidly, between midnight and 3:14 a.m. (GMT) on February 10. Again, the precise number was as close to 50,000 as the scan would allow, to ensure a like-for-like comparison.
This scan returned a CTM score of 46.81, far above the usual range for organic traffic and comparable with some of the most heavily gamed hashtag campaigns the DFRLab has encountered hitherto, though it paled in comparison to the pro-Modi effort.
Just like #TNwelcomesModi, #GoBackModi was heavily pushed by a small number of high-volume accounts that posted hundreds of times an hour. Unlike #TNwelcomesModi, these accounts were still not suspended at the time of drafting.
The most active was @PhillyTdp, which posted on #GoBackModi 2,179 times as the hashtag took off — a staggering one tweet every 5.3 seconds for over three hours.

According to an analysis performed using the open-source tool Twitonomy, the same account posted 2,453 times on February 10; 98 percent of all its tweets since February 9 were retweets.

Other accounts were similarly hyperactive. The second most active, @nritdpusa, posted 1,899 times in three hours, or roughly one tweet every 6 seconds.

Again, an analysis using Twitonomy confirmed that the account launched a massive series of posts on February 10. In total, it posted 2,459, almost exactly the same number as @PhillyTdp launched.

The third most active account, @ap_cbn, posted comparatively less often but still registered 1,194 tweets in just over three hours on the hashtag #GoBackModi on February 10, for an average rate of one post every 10 seconds.

An analysis using Twitonomy recorded that this account posted 1,807 times on February 10 and that 96 percent of its posts since December 27 were retweets.

These individual bots, if anything, were even more prolific than their pro-BJP rivals. Overall, however, the scan of #GoBackModi revealed a larger number of lower-activity accounts. In total, the 50 most active accounts tweeting #GoBackModi generated 16,325 tweets during the three-hour scan — far fewer than their rivals but still far more than any human user could be expected to have done.
Overall, the nearly 50,000 tweets in the #TNwelcomesModi scan were posted by just 891 accounts, while the nearly 50,000 tweets in the #GoBackModi scan were posted by 7,394 accounts.
By any measure, #TNwelcomesModi saw a much more aggressive attempt to make the hashtag trend from a much smaller user base.
Appendix: Coefficient of Traffic Manipulation (CTM)
The CTM is designed to compare Twitter traffic flows, to see which appear to have been significantly manipulated. The CTM was originally published by the Oxford Internet Institute’s Computational Propaganda project.
The CTM takes three numerical values from a given Twitter flow: the percentage of traffic that is made up of simple retweets; the percentage of traffic generated by the 50 most active accounts; and the average number of posts per user in the flow.
These values can be extracted from Twitter’s data by accessing the API or by using a range of proprietary tools, such as Sysomos or Crimson Hexagon.
The CTM is expressed by the following equation, in which R is the percentage of retweets, F is the percentage of tweets from the 50 most active accounts, and U is the average number of posts per user:
CTM = (R/10) + F + U
In the case studies above, #GoBackModi consisted of 73.9 percent retweets, according to Sysomos. The 50 most active users generated 32.65 percent of all traffic. The 49,538 tweets in the scan came from 7,394 accounts, for an average of 6.7 tweets per user.
#GoBackModi CTM:
7.4 + 32.95 + 6.7 = 47.05
#TNwelcomesModi consisted of 69.7 percent retweets. The 50 most active users generated 61.2 percent of all traffic. The 49,727 tweets in the scan came from just 891 accounts, for an average of 55.81 posts per user.
#TNwelcomesModi CTM:
6.97 + 61.2 + 55.81 = 123.98
These figures can be compared with a series of earlier traffic flows that the DFRLab analyzed in detail. Three were primarily organic and not targeted by large-scale planned amplification: #4thofJuly, the word “Davos” during the World Economic Forum in the Swiss resort of the same name, and the initial traffic on the word “covfefe,” tweeted apparently in error by U.S. President Donald Trump. All of these scored a CTM of less than 12.
The other three were heavily gamed by a combination of human users and bots, as the DFRLab reported at the time. One example came from Poland, one from France, and one from the United States. All of these scored a CTM of more than 30.

#GoBackModi fit among the heavily gamed hashtags, well ahead of the U.S. example #DigDoug, and just behind the Polish #StopAstroturfing.
#TNwelcomesModi stood out from all previous examples, showing massively higher figures.

The CTM approach is not designed as a binary system to identify which accounts are bots and which are not. Rather, it is a relative measure, designed to situate a given traffic flow on the spectrum between organic and heavily manipulated content, regardless of whether the manipulation came from bots, humans, or any combination of the two.
Using this method, however, it is possible to compare different traffic flows according to clear and measurable characteristics. The DFRLab will continue to scan Twitter for manipulated traffic in the months ahead.
Follow along for more in-depth analysis from our #DigitalSherlocks.