DFRLab launches the 2024 Foreign Interference Attribution Tracker
FIAT dashboard documents allegations of foreign malign information operations in the 2024 US general election
DFRLab launches the 2024 Foreign Interference Attribution Tracker
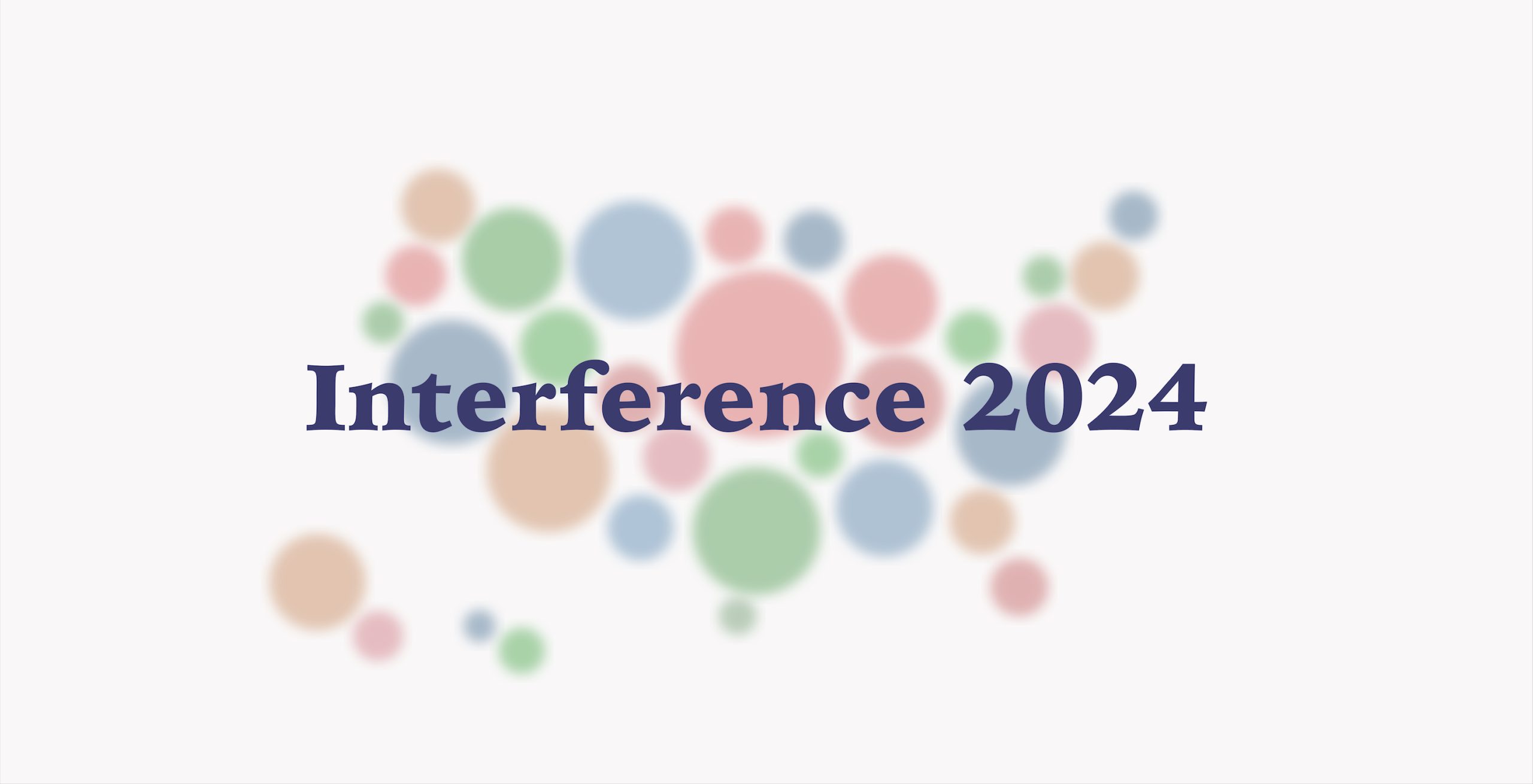
Today, the DFRLab released its 2024 Foreign Interference Attribution Tracker (FIAT), an interactive, open-source database cataloging attributions of foreign malign influence and interference regarding the November 2024 US general election. Available online at interference2024.org, FIAT categorizes relevant allegations of foreign malign influence and assesses their credibility and objectivity, as well as the evidence cited, their transparency, and overall impact.
Recent US government efforts to disrupt foreign malign information operations interfering in the 2024 US general election have garnered significant attention during the current election cycle, but these cases represent only a small share of suspected foreign interference documented since the November 2022 midterm elections. At the time of launch, FIAT has documented more than 40 cases of foreign malign influence and interference; the number of cases will undoubtedly grow in the weeks ahead.
Claims of foreign interference during the 2024 US election cycle have been incredibly diverse in their sources, their favored methodologies, and the objectivity of their analysis. Their volume and complexity can also be vexing for policymakers and journalists working to contextualize and respond to them.
We have designed FIAT will help address these challenges. Overall, FIAT has four goals:
- Provide an independent and reliable record of foreign malign influence and interference
- Build public attribution standards
- Serve as a resource for stakeholders about the evolving threat
- Help increase public resilience against future foreign influence and interference efforts, especially in online spaces.
The 2024 edition of FIAT builds on the success of our FIAT 2020 tool and begins to build a public understanding of how foreign influence targeting US elections over time. As in the previous presidential election cycle, DFRLab experts will regularly update FIAT as further allegations of foreign interference in the 2024 election become public. We will also implement additional functionality to the tool in order to build an enduring resource for the research community, policymakers, the media, and the general public.
Initial observations
Based on the more than 40 attributions of foreign malign influence and interference documented as part of this project, the DFRLab is already drawing insights into the timing and impact of these attributions. Among our initial observations:
- US government and intelligence agencies announcements/actions against foreign threat actors from China, Iran, and Russia accounted for almost half of the 40+ attributions. These include periodic pre-election warnings about the threats of foreign malign influence and interference from the Office of the Director of National Intelligence and actions taken against identified actors by the US Department of Justice.
- Utilizing inauthentic social media accounts to amplify propagandistic, divisive, and false content is the most popular method utilized by foreign threat actors. About half of attributions identified at the time of publishing included the use of “sockpuppet” accounts in malign influence operations targeting US audiences.
- US-based social media platforms continue to provide evidence-driven attributions of foreign interference. Similar to what we observed in 2020, US-based platforms continue to provide attributions of foreign interference that is more evidence-driven in comparison to attributions by US agencies and officials, some of which rely on confidential evidence.
- Foreign interference attributions regarding China, Iran, and Russia have highlighted each actor’s established tactics, techniques, and procedures (TTPs). Chinese actors targeting local elections and US audiences with conspiracy theories and divisive narratives about sensitive social and political issues through fake accounts on social media as part of an influence campaign dubbed “Spamouflage.” Iranian actors conducted cyber operations targeting presidential campaigns and launch influence campaigns targeting US audiences with content about the escalating conflict in the middle east. Russian actors deployed narratives that undermine confidence in the elections, democracy, and support for Ukraine through fake news websites attributed to its Doppelganger operation.
How we select individual cases
For inclusion in our 2024 database, cases must fulfill three criteria first developed for our 2020 FIAT project.
First, cases must involve allegations of foreign interference by primarily digital means. The Australian Government Department of Home Affairs defines foreign interference as efforts by a foreign actor that is “coercive, corrupting, deceptive, or clandestine” in nature, distinguishing it from more overt forms of foreign influence, such as lobbying and public diplomacy. By focusing expressly on digital activity, this definition denotes a range of interference activities—including disinformation, media manipulation, and cyber intrusion—that are conducted by foreign actors to affect political outcomes. As such, the database does not include day-to-day publishing activities by state media actors; to do so would fill the database with anti-US propaganda from RT or Xinhua, for example, rather than instances of specific campaigns to interfere with the US election.
Second, cases must be novel. A novel case is one which involves a fresh allegation of foreign interference or one that reveals new evidence of a foreign actor reinvigorating a pre-existing campaign. A novel case can also be one in which significant newsworthiness is attached to the individual or organization making the claim. For example, claims made by a current or former president would be considered newsworthy regardless of the evidence presented, while an op-ed or report by a mid-level US official is only novel if it contains previously undisclosed information.
Third, cases must be relevant to the 2024 US election. Cases included in the database involve alleged instances foreign interference intended to influence voting behaviors, denigrate particular candidates, or engage in political or social issues of direct relevance to the 2024 general election. As such, cases in the database span from the November 2022 US midterm election through November 2024.
How cases are scored
In order to assess and contextualize foreign interference claims properly, FIAT 2024 employs two distinct measurements: Breakout Scale and Attribution Score.
The Breakout Scale,a comparative model designed by former DFRLab Nonresident Senior Fellow Ben Nimmo in 2020, categorizes each case’s reach and potential impact based on its spread across platforms, communities, and media types.
The Breakout Scale is comprised of six numerical categories, with higher numbers representing more significant instances of a breakout occurring:
- Category One: The case is confined to one platform with no breakout (i.e. the messaging does not spread beyond the community at the insertion point).
- Category Two: The case is confined to one platform but there is breakout OR is on many platforms with no breakout (insertion points on multiple platforms, but messaging does not spread beyond them).
- Category Three: The case has insertion points and breakout moments on multiple platforms, but it does not spread onto mainstream media.
- Category Four: The case features cross-medium breakout beyond social media. It is reported by mainstream media as embedded posts or as reports.
- Category Five: Celebrity amplification or endorsement.
- Category Six: The case triggers a policy response or some other form of concrete action, or it includes a call for violence.
Attribution Score, meanwhile, is a framework of eighteen binary statements (true or false) intended to assess foreign interference claims made by governments, technology companies, the media, and civil society organizations. If a statement is deemed applicable, a point is awarded. If a statement is deemed inapplicable or irrelevant, no point is awarded. This scoring system was originally developed for FIAT 2020 and is based on the experience of DFRLab experts in assessing—and making—such allegations. It is also based on a review of work produced by the wider disinformation studies community, and particularly resources compiled by attribution.news.
The Attribution Score is composed of four subsections:
Credibility
- The source of the attribution does not have a direct financial interest in a certain attribution outcome.
- The source of the attribution has a diversified and transparent funding stream.
- The source of the attribution does not strongly endorse a specific political ideology.
- The source of the attribution is in no way affiliated with a political campaign.
- The source of the attribution has not previously promoted mis- or disinformation.
Objectivity
- The attribution avoids using biased wording. The attribution avoids high-inference or emotive language.
- The headline accurately conveys the content of the attribution.
- The attribution clearly distinguishes factual information from argumentative analysis.
Evidence
- The attribution provides a clear illustration of the methods, tactics, and platforms involved in the alleged information operation.
- The attribution contextualizes the engagement with, and impact of, the alleged information operation.
- The attribution identifies actors and states allegedly responsible.
- The attribution clearly explains the strategic goal and rationale of the actors who conducted the alleged information operation.
- The attribution relies on information which is unique to, or can only be procured by, the relevant actor. (e.g. classified information for U.S. federal agencies, back-end/developer information for technology companies)
Transparency
- The attribution provides open access to a dataset or archived links of alleged assets.
- The attribution methodology is clearly explained.
- The attribution is replicable through open-source evidence.
- The attribution acknowledges relevant limitations or mitigating factors in its assessment.
- The attribution has been corroborated by a third party or independent investigation.
The 2020 edition version of the FIAT database also featured a metric called “Attribution Impact,” which aggregated Facebook, Reddit, and Twitter engagements to approximate the prevalence of a given allegation in the American news cycle. Due to the shutdown of Meta’s CrowdTangle tool and increased restrictions on platform APIs, this measure could not be replicated for the new dataset.
Instead, FIAT 2024 is supplemented with data from X – formerly Twitter – gathered from an API provided by the social media monitoring tool Meltwater Explore. This data was queried using a combination of a country term and an interference term. For example, the query for Russian interference contains relevant variants of a country term, such as “Russia,” “Russian,” “Putin,” or “Kremlin,” while an influence term, involves variants of words including “interference,” “influence,” and “propaganda.” Using Meltwater Explore, the DFRLab collected X data from January 1, 2024 through the end of November 2024 for Russia, China, and Iran. Posts are aggregated by the count posted per day for each country and graphed on the tracker.
The DFRLab will share additional findings from the FIAT 2024 dataset over the course of the election cycle and beyond. Readers can evaluate the data themselves by visiting interference2024.org.
The core FIAT research and technical team is composed of Max Rizzuto, Dina Sadek and Meredith Furbish, under the oversight of Emerson T. Brooking. The tool was designed by Maarten Lambrechts. Special thanks to DFRLab Young Global Professional Julien Fagel for providing additional support for this project.
Cite this case study:
Dina Sadek, Meredith Furbish and Max Rizzuto, “DFRLab launches the 2024 Foreign Interference Attribution Tracker,” Digital Forensic Research Lab (DFRLab), October 23, 2024, https://dfrlab.org/2024/10/23/dfrlab-launches-fiat-2024/.