The evolving role of AI-generated media in shaping disinformation campaigns
A methodological examination of how malicious actors exploit visual generative models
The evolving role of AI-generated media in shaping disinformation campaigns
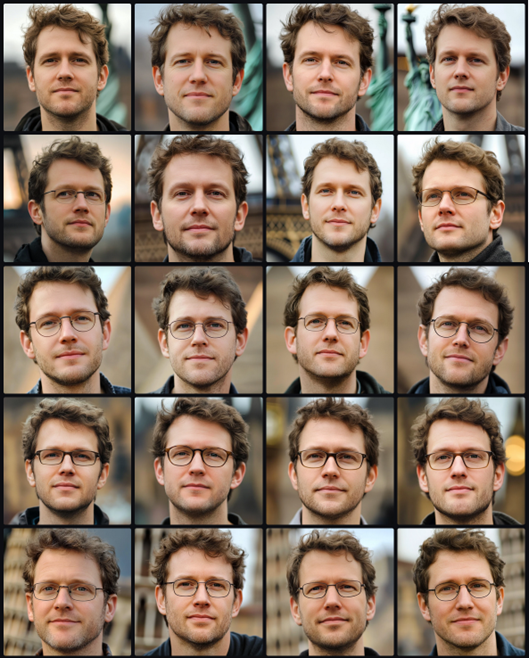
Banner: Midjourney-generated images of a subject in various settings. In descending order, the backgrounds show the Statue of Liberty, the Eiffel Tower, the Pyramids of Giza, Big Ben, and the Leaning Tower of Pisa. (Source: DFRLab via Midjourney)
Malign actors are exploiting the rapid evolution of generative artificial intelligence (GAI) to create synthetic media that enhances their existing methods of operation. This developing trend is transforming visual content creation for deceptive purposes and possibly complicating the identification of AI-generated media. Although experts and users can often detect revealing patterns in such content, recent cases of realistic AI-generated images and deepfakes from different parts of the world highlight the ease with which bad actors can create and spread deceptive visual content. In this report, the DFRLab focused on the role of visual generative models in creating profile pictures to understand AI tools’ progression and examine its use in future influence operations.
Generative artificial intelligence, generative adversarial networks, and diffusion models
GAI, a technology that can create different types of content based on training data, has existed for decades. However, the introduction of generative adversarial networks (GAN) marked a turning point, as these advanced machine learning applications significantly enhanced and democratized the creation of synthetic media that may be photorealistic and indistinguishable from labor-intensive human-made content.
Moreover, advancements in multi-modal AI systems, the base for tools like Midjourney, Dall-E, and Stable Diffusion, and its cheap or free access provided users an easy route to generate images and videos from descriptive text inputs. In this examination, we refer to such systems and tools under the umbrella term diffusion models.
This Person Does Not Exist
Before the widespread accessibility of diffusion models, researchers documented the rise and use of the generative adversarial network (GAN), specifically to create high-resolution synthetic faces, which were widely documented for their use in profile picture generation by inauthentic accounts in various online operations, ranging from commercial activities to political propaganda. Yet, as salience surrounding these tactics proliferated, researchers and users with a discerning eye became aware of how to spot the common tell-tale errors and patterns that exposed the provenance of such images.
Debuting in 2019, ThisPersonDoesNotExist.com, a project based on the then-popular StyleGAN architecture (and later StyleGAN2), saw widespread use in deceptive campaigns. As the name would suggest, ThisPersonDoesNotExist was trained on a dataset of human figures and could generate unique, synthetic images of non-existent human subjects.
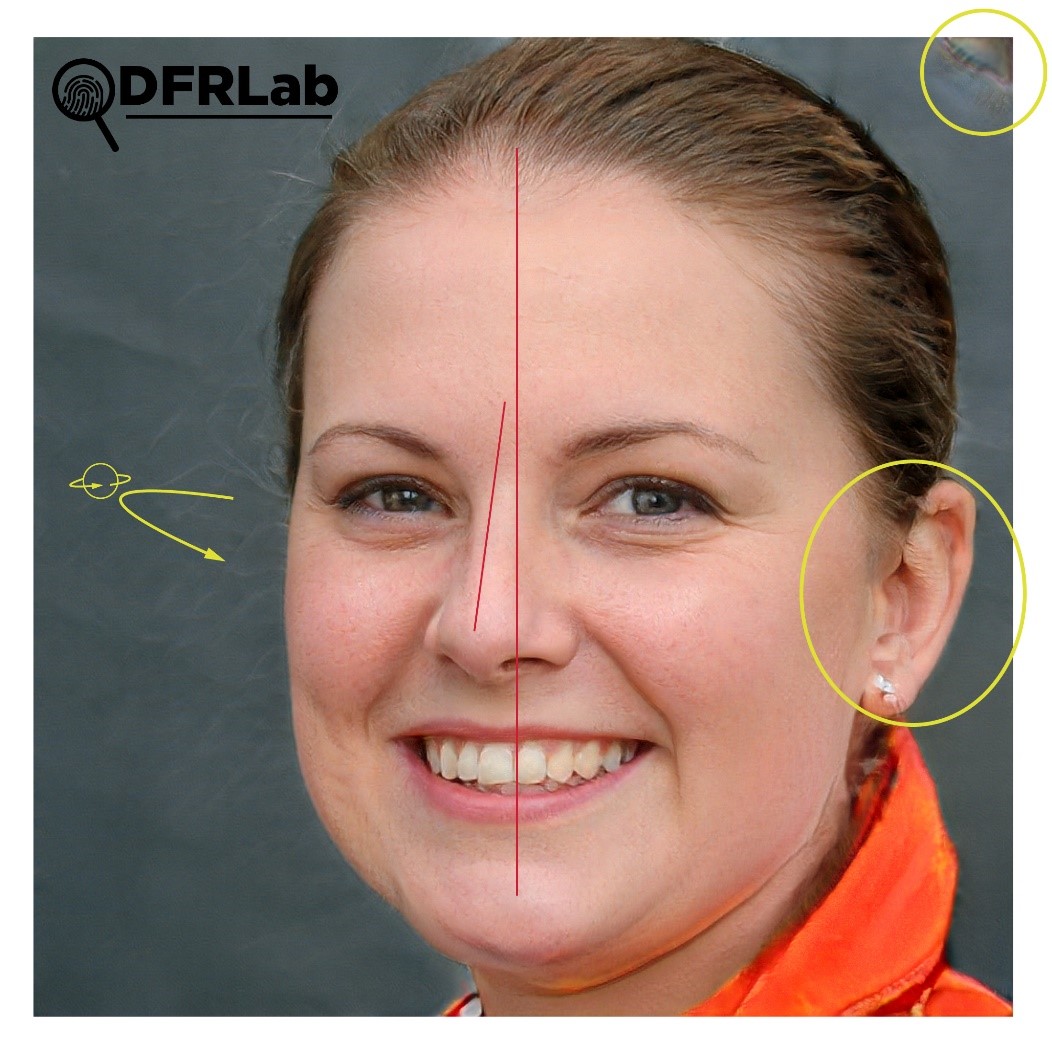
Unlike diffusion models, the scope of the subject matter which a single GAN can produce is limited to the subjects included in the model’s training data. Spin-off projects such as TheseCatsDoNotExist, ThisCityDoesNotExist, and ThisRentalDoesNotExist exemplify the technology’s reliance on large and accessible training datasets, the limited scope of the subjects it can produce, and the compositional uniformity of the generated images. Furthermore, these image generation tools offered no artistic control over the subjects they produced and simply served images at random when the webpage was refreshed.
Still, ThisPersonDoesNotExist became the preferred resource for adding credibility to otherwise lifeless sockpuppet accounts. As this tactic became increasingly prevalent, the media extensively reported on the technology and existing detection techniques.
The latest generation of GenAI for image synthesis excels in many areas where early GAN models fell short. In recent years, the prevalence of diffusion models has surpassed GAN-generated images, rendering moot many of the so-called “inoculation efforts” suggested by media and civil society, for example, exposing internet users to the exploitive capabilities of certain deceptive tactics and techniques to the extent that an acquired sense of salience about the capabilities might protect them from future deceit.
GAN vs diffusion models vs multi-modal models
Unlike GANs simply vending images to users, modern diffusion-based tools afford seemingly limitless artistic control. The text-to-image format of image generation allows for a great deal of control when defining the subject of an image. Some diffusion models, such as Midjourney, allow users to tweak and tune even the most granular details of an image and its subjects; these subtle details were often the giveaway when attempting to discern the authenticity of a GAN image.
For instance, GAN-generated headshots typically struggle with generating inorganic objects that demand symmetry, such as glasses or jewelry. With Midjourney, not only can glasses be rendered with accuracy and symmetry, but they can also be modified or removed entirely. This is a fixture of the model’s instruction-based approach, which affords users a great deal of freedom and specificity in defining their subject.
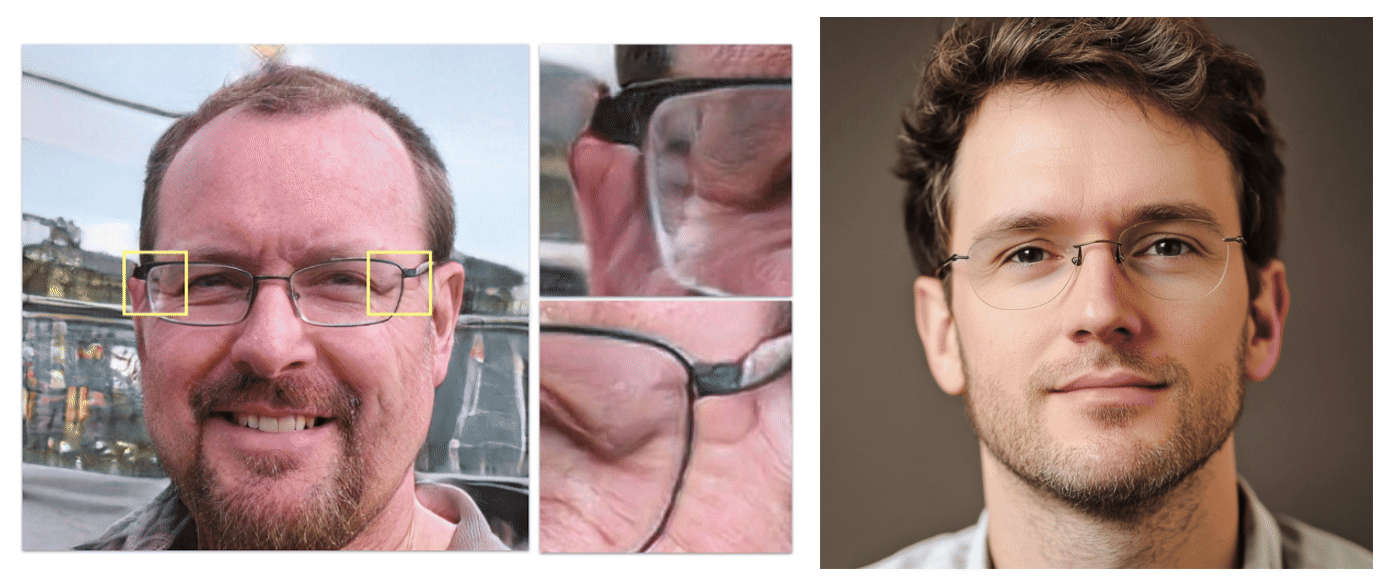
GAN-generated headshots also struggled to create cogent backgrounds and frequently hallucinated when rendering the settings behind the subjects. Not only do these diffusion models excel in creating believable settings, but they also afford users the ability to curate backgrounds to their liking.
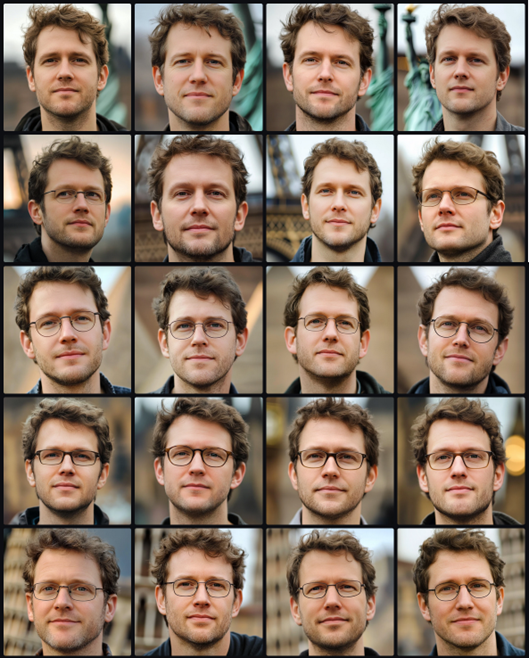
GAN-generated images are created using two different networks: a generator network tasked with generating fake images, which competes with a discriminator network which compares the generated images to the training data. When the generator is able to produce an image which satisfies the discriminator, the image generation is complete. Diffusion-generated images, however, are created from random noise and then iteratively denoising the data until the image emerges, which enables the model to refine the image during generation without the need for retraining.
Unlike GAN images, diffusion-generated images are not limited to the narrow subject matter of just one training dataset. Diffusion models can generate content in a text-to-image format for any subject on which they have been trained.
For example, when prompted to generate an “image of a researcher at the Atlantic Council’s Digital Forensic Research Lab,” Midjourney produced the below image:
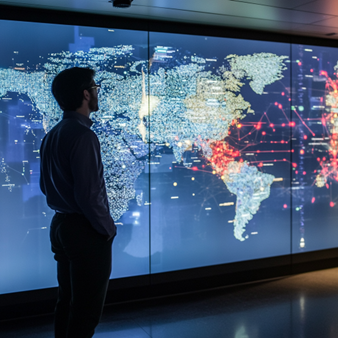
The model recognized the DFRLab as an institution, placing a human subject in business attire in an indoor setting in front of the kind of displays one might find at a DC-based foreign policy think tank. To generate this image, Midjourney broke down the prompt into tokens or fragments and proceeded to contemplate each component piece; weights assigned to each token directed the model to gradually remove noise from an array of random data to produce an image based on the context provided and the relevant data available in its training dataset.
Multi-modal models build upon the diffusion technique but expand upon the provided context (the prompt) by incorporating information from a variety of modalities (text, in addition to audio-visual data). This allows the model to better interpret the users’ intent and bridge visual and textual domains when reasoning prior to generating an image.
When a similar prompt was posed to a multi-modal model, Open AI’s ChatGPT-4o, the output reflected dramatically improved results, including the Atlantic Council’s branding, typeface, and color scheme, and depicted the subject in an office setting seemingly doing the sort of security research for which the DFRLab is known. The multi-modal model excelled in this case because of its ability to reason after the instructions were delivered and before the image was generated. This post-training reasoning allows GPT-4o to provide greater context to support the otherwise sparse prompt.
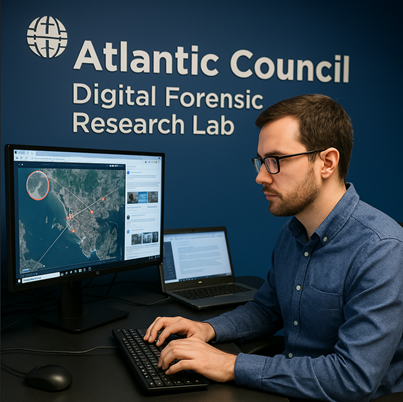
Case studies: Multi-modal and diffusion-generated images
Pro-UAE accounts Target COP28
Before the United Nations (UN) COP28 climate summit, held in late 2023 in the United Arab Emirates (UAE), an inauthentic network on X used a mix of GAN-generated and possibly multi-modal profile pictures to promote the president of the summit, Sultan Al Jaber. The DFRLab documented instances that underscore the role of synthetic images in the campaign, signifying early adoption of the latest generation of AI tools.
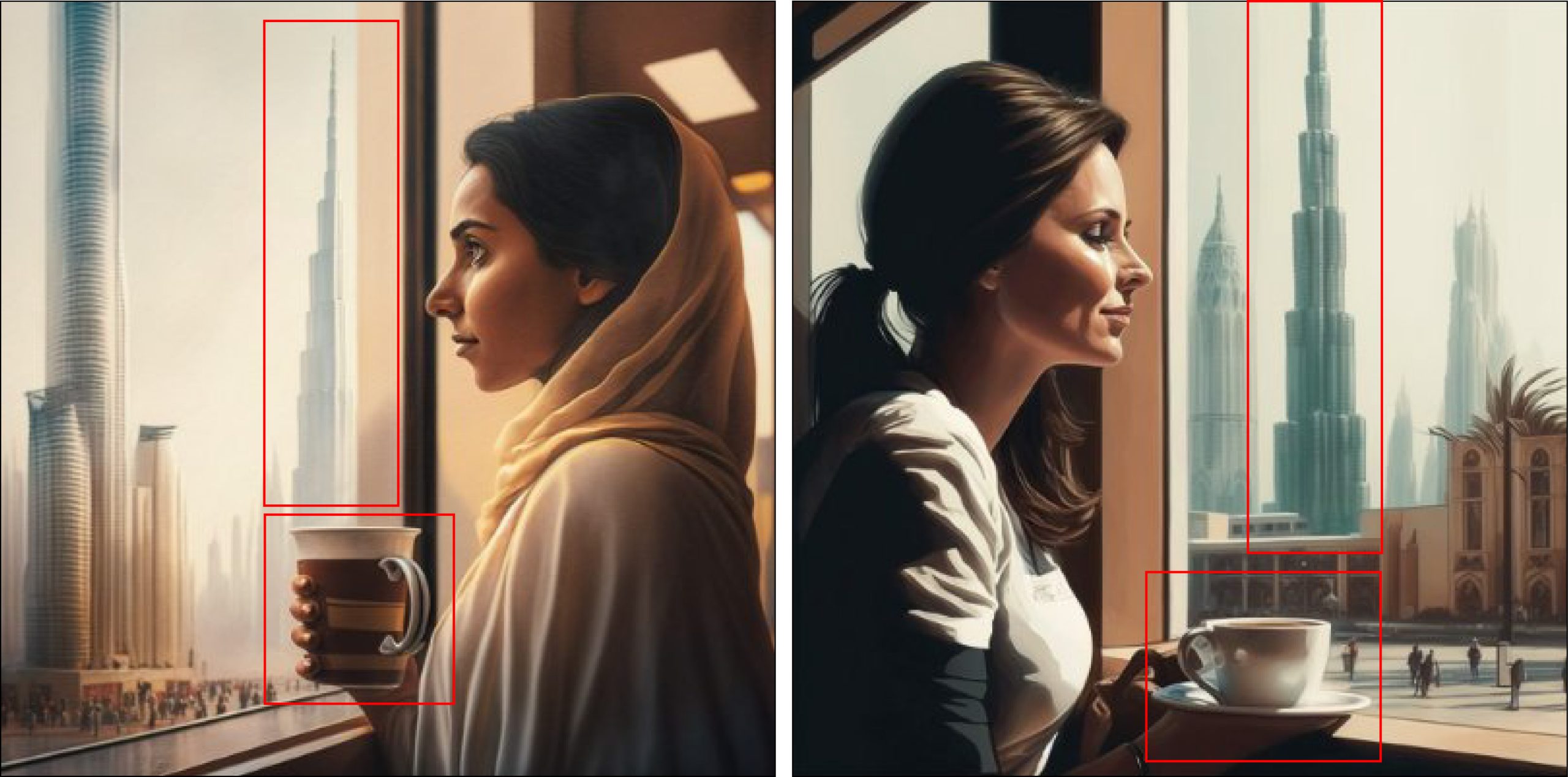
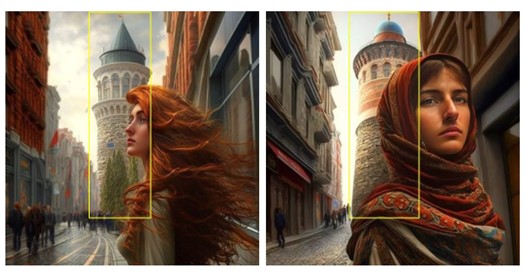
The above examples also demonstrate that some amount of provenance can be derived by identifying themes across images and profiles. The above images, which had a uniform theme surrounding landmarks and female subjects, were posted across various accounts. This identification strengthens the hypothesis that the observed assets originated from the same source or were generated using the same prompt, possibly at the same time.
To further examine the thematic pattern of the generated images uncovered in the pro-UAE network, we experimented with how various models react to variations of a templated prompt, recreating one manner in which the profile pictures may have been generated.
The table below shows how popular image generation models react when prompted with: “Image of a(n) [insert nationality] woman standing in front of a monument.” We found that the models tended to assume that nationality was a reflection of the subject’s location.
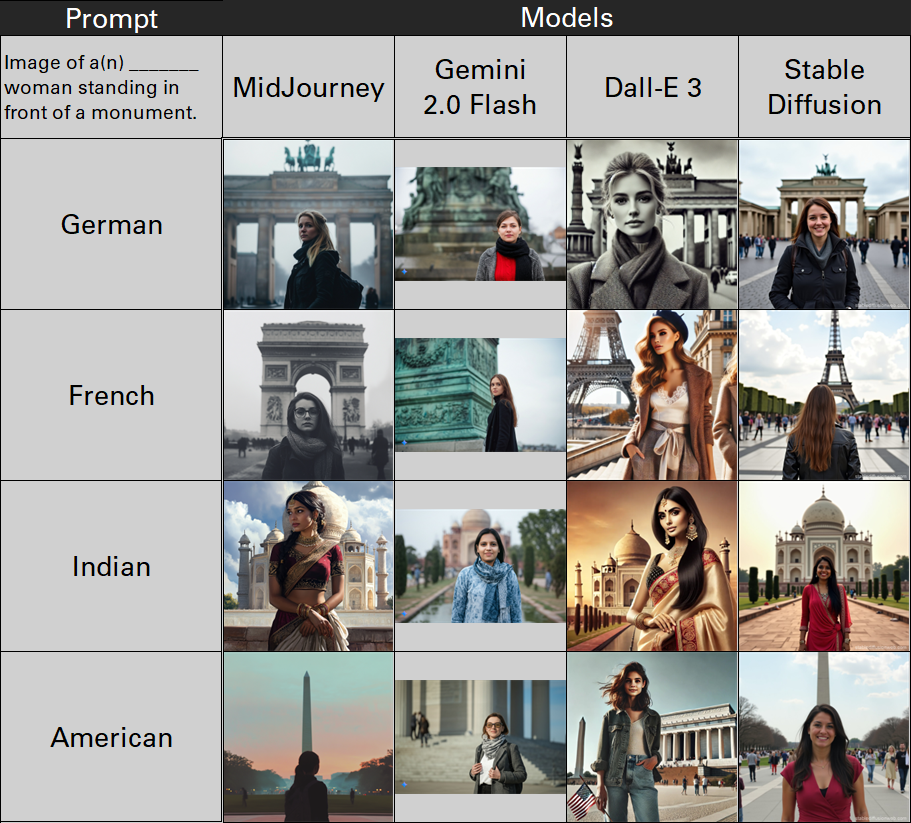
Though it may offer limited insights, this observation does provide a view into how AI-generated images can be used to expedite content creation by using images derived from the same prompts and potentially the same formats, which aligns with previously observed behavior in which speed is favored over quality when constructing an inauthentic network of accounts.
Inauthentic Facebook accounts undermine Moldova’s pro-European government
An inauthentic Facebook ad campaign used to undermine Moldova’s pro-European government and promote the country’s pro-Russia party served as another example of the developing use of multi-modal models. This campaign used tools such as DALL-E 2 to create synthetic profile pictures that not only showed faces but also included upper bodies to make them appear more realistic.
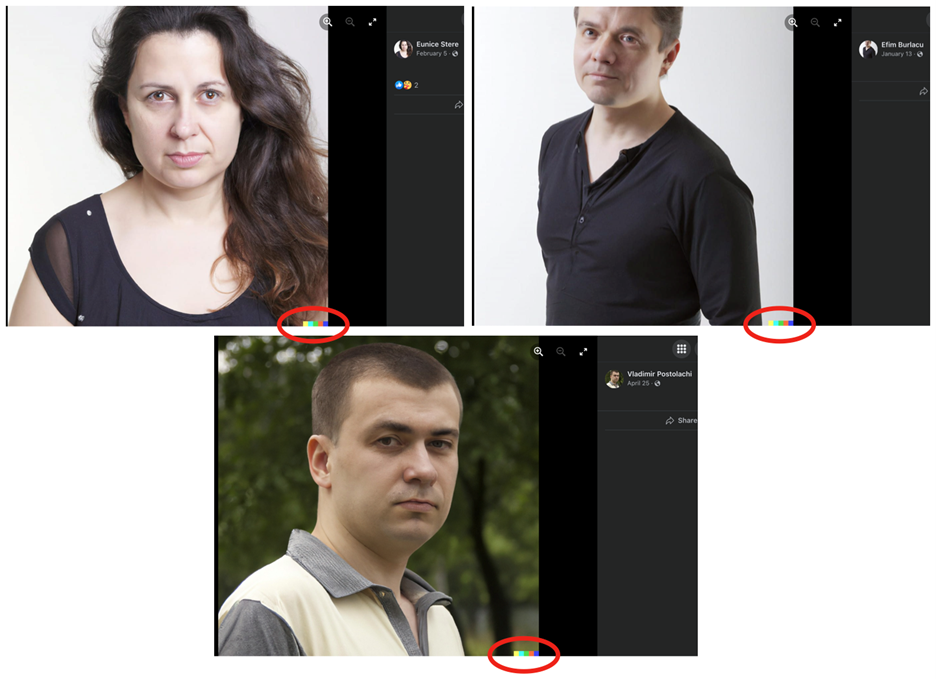
STOIC: Inauthentic campaign targets Americans and Canadians
A DFRLab investigation into a campaign attributed to the Israeli government revealed an attempt to create seemingly realistic X profiles via diffusion-based synthetic profile pictures. One of the network’s inauthentic campaigns, which targeted US and Canadian politicians and users, used GAN-generated images. However, in another operation likely tied to the same group, more than 100 accounts in the network employed lifelike profile pictures created using diffusion models, highlighting an evolution of tactics.
The DFRLab and other researchers identified and analyzed the campaign in early 2024. Meta and OpenAI later attributed the campaign to the Israeli marketing firm STOIC. Then, reporting by the New York Times, Ha’aretz, and FakeReporter revealed that Israel’s Ministry of Diaspora Affairs had funded the campaign.
The profile pictures utilized in the campaign represented a wide variety of ethnicities, including young males and females, and could appear as normal pictures from afar. However, a closer look revealed unusual similarities, inaccuracies, and details that seemed unnaturally perfect.
Some male subjects in the STOIC network appeared across multiple assets with subtle variations. This led the DFRLab to surmise that it was likely Midjourney that was used to create the images. As shown in the above table, which is related to variance in the setting of a given subject, Midjourney allows for the creation of new images with subtle variations of a given character. Identifying similar-enough characters within the network could be used to draw conclusions about the provenance of the images.
Additionally, because some of these tools generate a set of images at a time, four in the case of Midjourney, identifying AI-generated doppelgangers across accounts may allude to the existence of other assets which have not yet been observed. For instance, for the examples below, which contain fewer than four doppelgangers, it would not be unreasonable to surmise that the unobserved images may have already been or will be used on unidentified profiles.
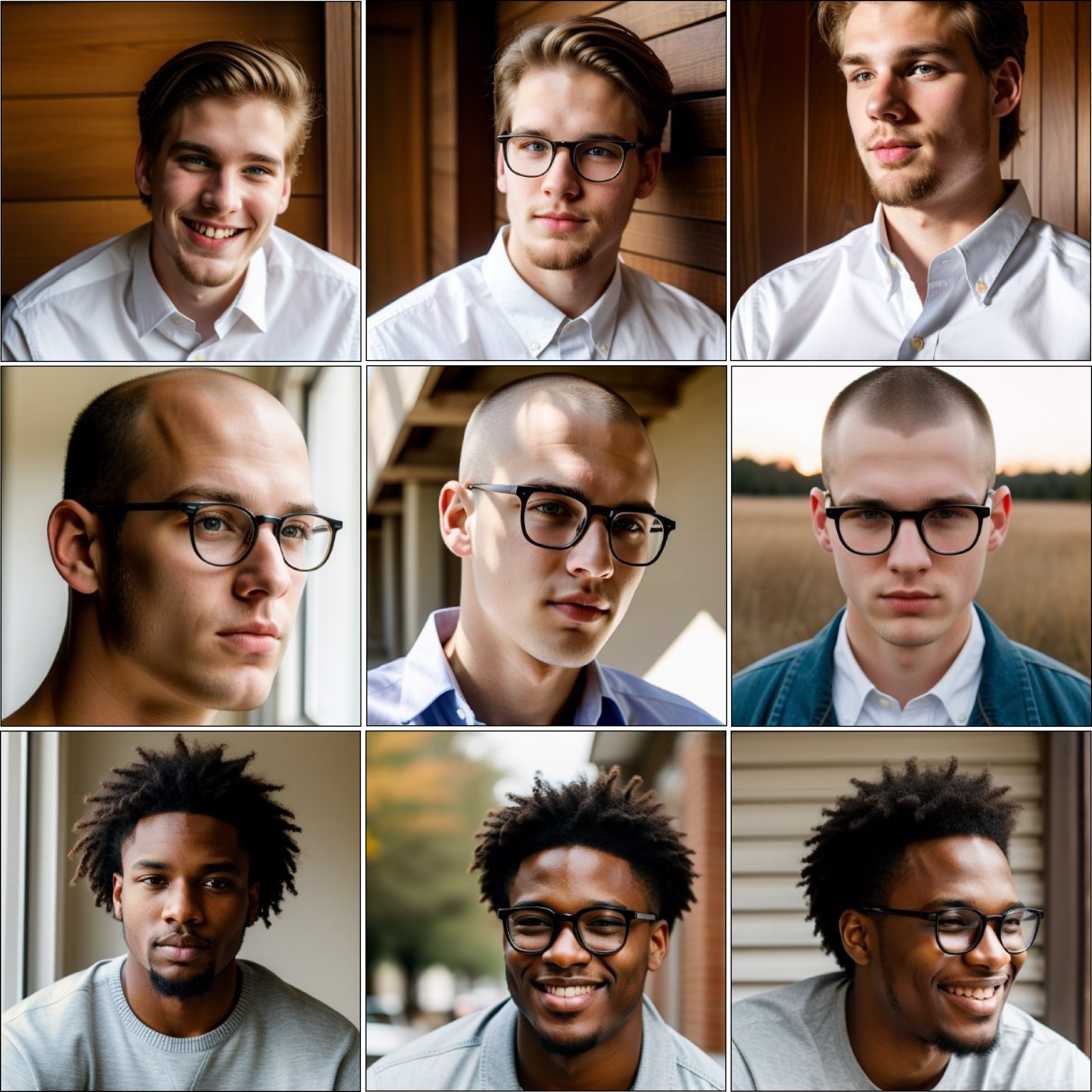
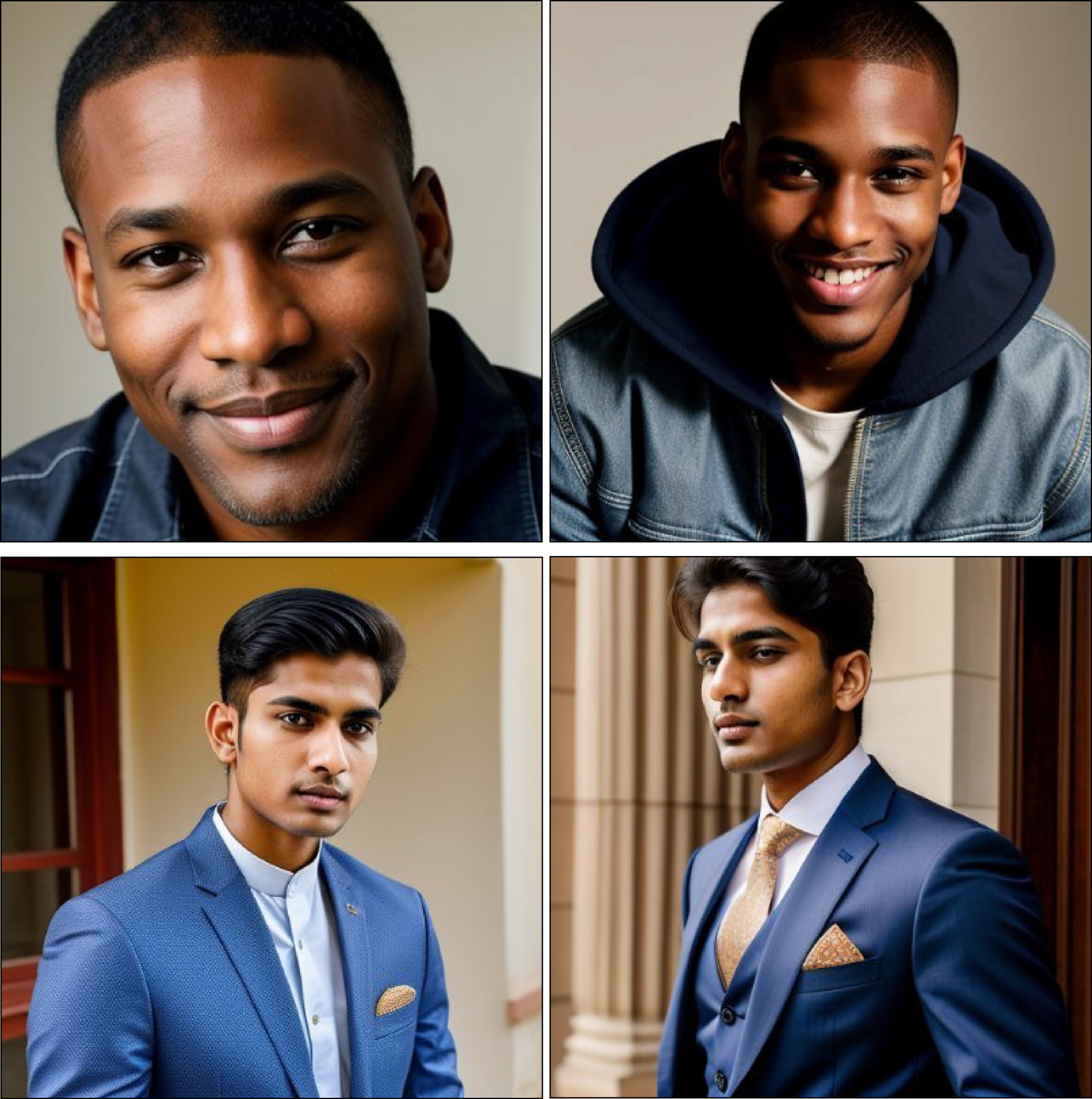
Pro-Israel sockpuppets on X impersonate Americans and Canadians
In another example, the DFRLab identified a pro-Israel network on X that used both GAN-generated and hyperrealistic profile pictures to impersonate Americans and Canadians. Though this network has no connections to STOIC, it appears to have utilized similar text-to-image models to generate the avatars used by the fake accounts, which had similar styles and indicators, such as misspelled text and studio-quality portraits with perfect lighting and smooth skin.
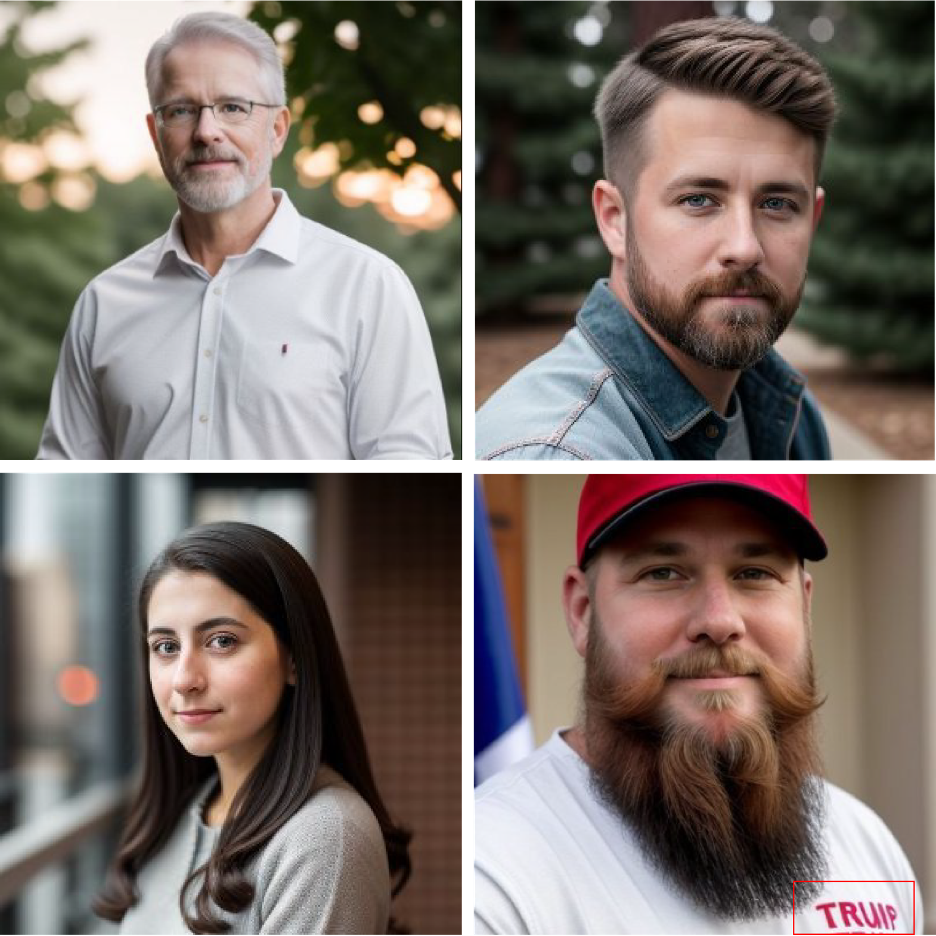
AI tools used in Kenya to discredit protesters and allege Russian connections
The text-to-image format opens a world of possibilities for those with the intent to deceive. This technology has been used to create deceptive images in various contexts beyond just profile pictures for inauthentic accounts. We have observed several diffusion-derived pictures used in politically motivated contexts.
For example, following protests in Kenya in the summer of 2024, the DFRLab identified an influence operation that used AI-generated images depicting young Kenyans protesting with Russian flags and same-sex protestors kissing in an effort to discredit the protests. Even though it does not appear that the photos were created to be perceived as real, images such as these are used “mainly to illustrate a given narrative with striking or symbolic images,” as noted by a VIGINUM report examining similar cases. This tactic exemplifies the developing trend of using AI tools to aid operations in different parts of the world.
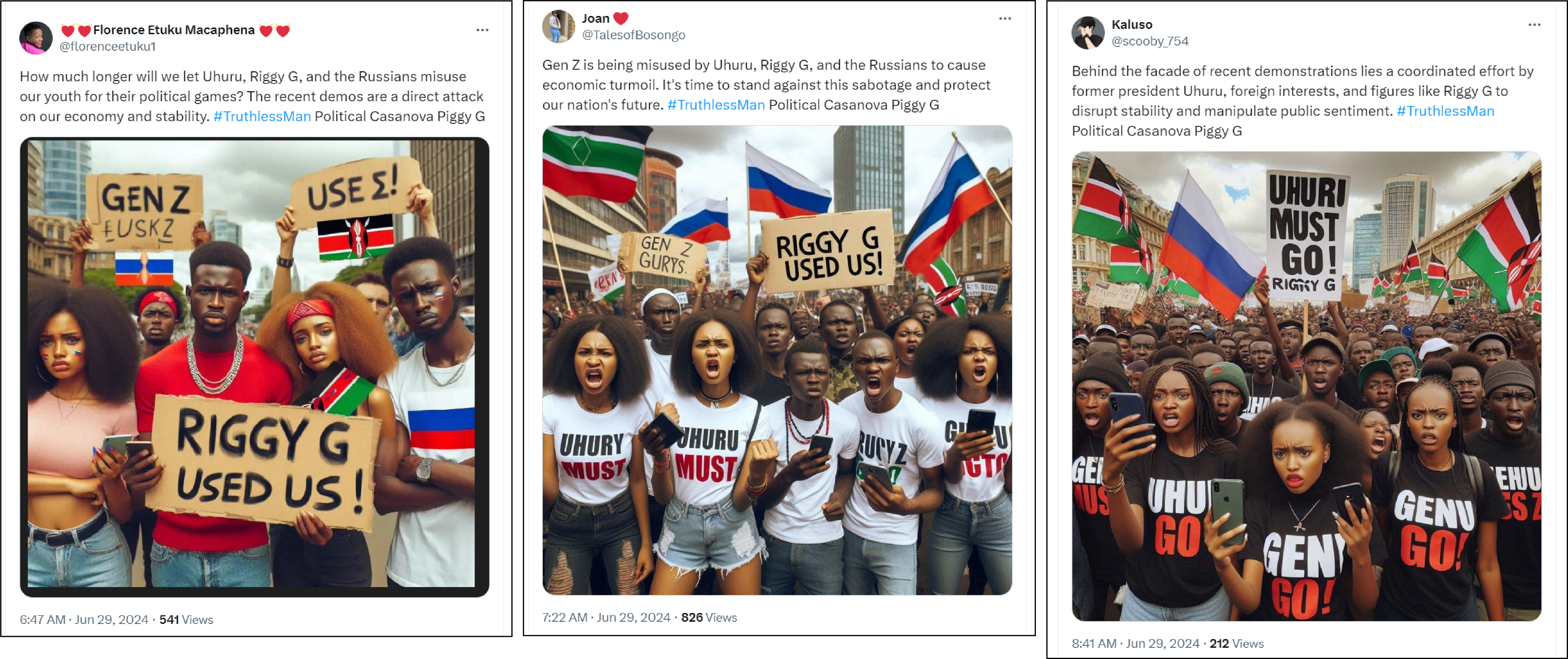
Detecting visual patterns
As text-to-image models improve, they will continue to present difficulties for researchers, journalists, and internet users who endeavor to discern the veracity of images online. While the latest models have nullified many of the detection techniques that were once central to identifying GAN-generated images, they produce their own artifacts, which are more subtle than GAN’s hallucinations but no less ubiquitous.
Images created by diffusion models have a somewhat uniform aesthetic. These images are often cinematic, appear with a painting-like quality, and consistently reflect high contrast and soft lighting.
For instance, many pictures used by the accounts in the prior examples could be deemed “uncanny” or “too perfect,” with studio-like lighting, textbook composition, and perfect symmetry, poses, and angles. Faces also had unrealistically uniform and clear skin textures, lacking subtle but common human imperfections.
Trained on social media and publicly available datasets that may disproportionately feature images of actors, models, or other conventionally attractive individuals, diffusion models often exhibit a bias toward certain aesthetic preferences. When generating images of people, idealized beauty norms are frequently apparent, with outputs resembling professional models. When instructed to deviate from these norms, the models tend to produce caricature-like figures rather than realistic alternatives. GAN-generated images of human subjects, specifically originating from StyleGAN2, excel in generating common human subjects as the GAN model was trained on images of human faces scraped from Flickr and, as such, reflect the crowd-sourced dataset of subjects.
Additionally, in cases where the backgrounds are not distinct or recognizable, the backgrounds in text-to-image derived pictures often appear generic and blurred, lacking verifiable environmental details. In the instruction-based approach of multi-modal models, this could be attributed to a lack of specific localization in a prompt, which leads to notably generic and featureless settings.
GAN-generated images of human subjects typically struggled to reproduce articles of clothing. As with accessories, such as jewelry or glasses, clothing was never the strength of the GAN models.
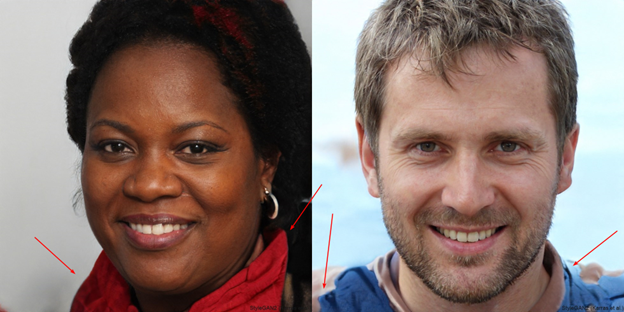
Although diffusion models have made progress in remedying the issues with clothing and fabrics, they still occasionally produce artifacts of their own in relation to certain materials. Diffusion-generated images featuring textiles with repeating patterns or defined textures are frequently inconsistent. Inconsistencies in thread patterns and overall asymmetry in the design may serve as cues that text-to-image models generated the image. This issue is less prevalent when generating finer textiles with smaller, more tightly woven threads, where the individual strands are less visible; however, the models struggle with uniformly patterned fabrics.
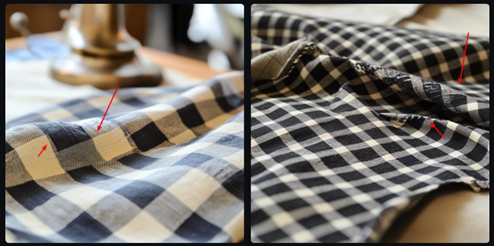
Previously, hallucinations were a far more prevalent and reliable method of identifying diffusion-generated images. The models tended to lose focus when tasked with generating uniform details or objects that require consistency, such as fence posts and windows. The produced images appeared to interpolate known features with imagined ones, and the resulting depiction was often a giveaway that the image was inauthentic. A notable example in 2023 involved a hallucinated image of an explosion near the Pentagon that went viral online. The image was shared by the verified account of the Russian state media outlet RT and by an inauthentic account, “Bloomberg Feed,” posing as a subsidiary of the Bloomberg brand.
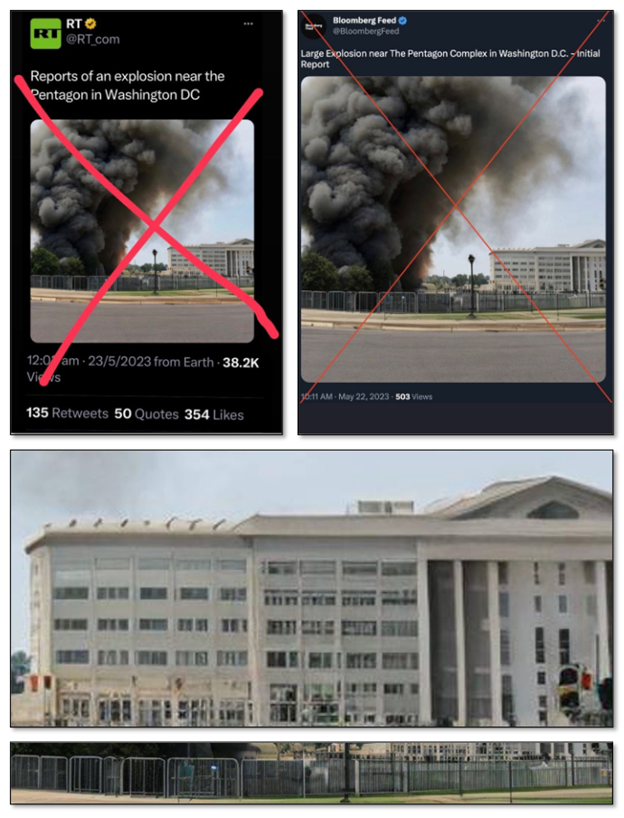
For human subjects, diffusion models are known to struggle when tasked with generating realistic hands, often producing anatomically implausible reproductions that deviate from the typical five-finger arrangement. The continued development of diffusion models has shown progress in this area, but as a means of detection, hands remain a good place for researchers to focus their attention.
Reflecting on the earlier STOIC example, although many of the images in the sample did not include clear errors or inaccuracies, some did. Such inaccuracies were commonly associated with issues rendering hands, text, and facial hair.
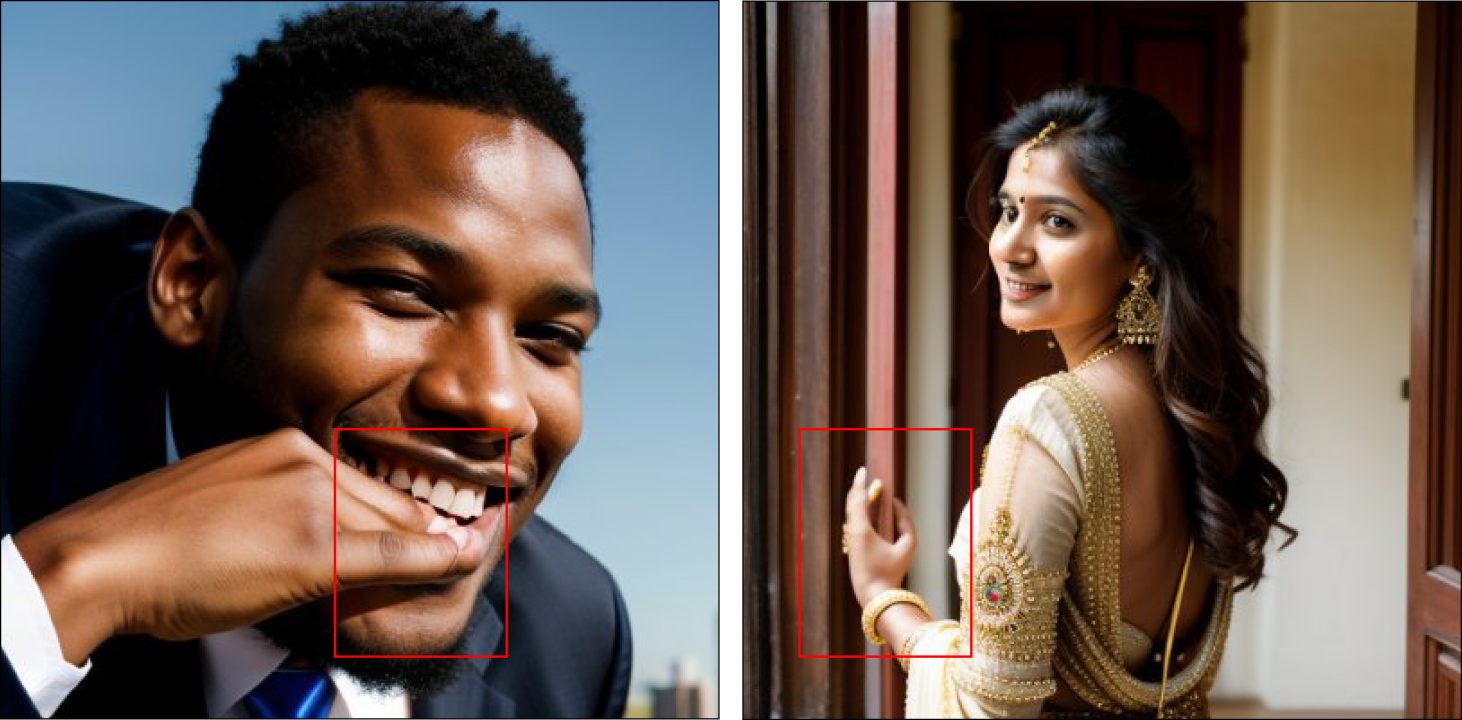
As the generation of human anatomy by diffusion models continues to advance and the hallucinations become ever more subtle, critical inspection will be required by researchers, journalists, and internet users alike. While misspellings in text continue to be a challenge for many models, developments have already been made to address this issue by Open AI’s ChatGPT-4o.
Deepfakes
Sophisticated synthetic AI-generated media also comes in the form of deepfakes. With this technology, malign actors create or alter visuals to depict individuals in imagined scenarios or performing actions that never occurred, often by swapping voices or faces.
Research shows that malicious actors in different parts of the world are using such tactics to support their activities. The types of synthetic content created often include crypto scams, pornography to attack women in politics and journalism, political propaganda, election deception, and attacks against politicians and leaders.
The DFRLab previously examined instances of deepfakes and AI-generated content across several platforms in Brazil’s 2024 municipal elections, following electoral rules banning unlabeled AI-generated content. Instances included deepfake pornography targeting female candidates in addition to the impersonation of prominent media figures to boost or undermine candidates.
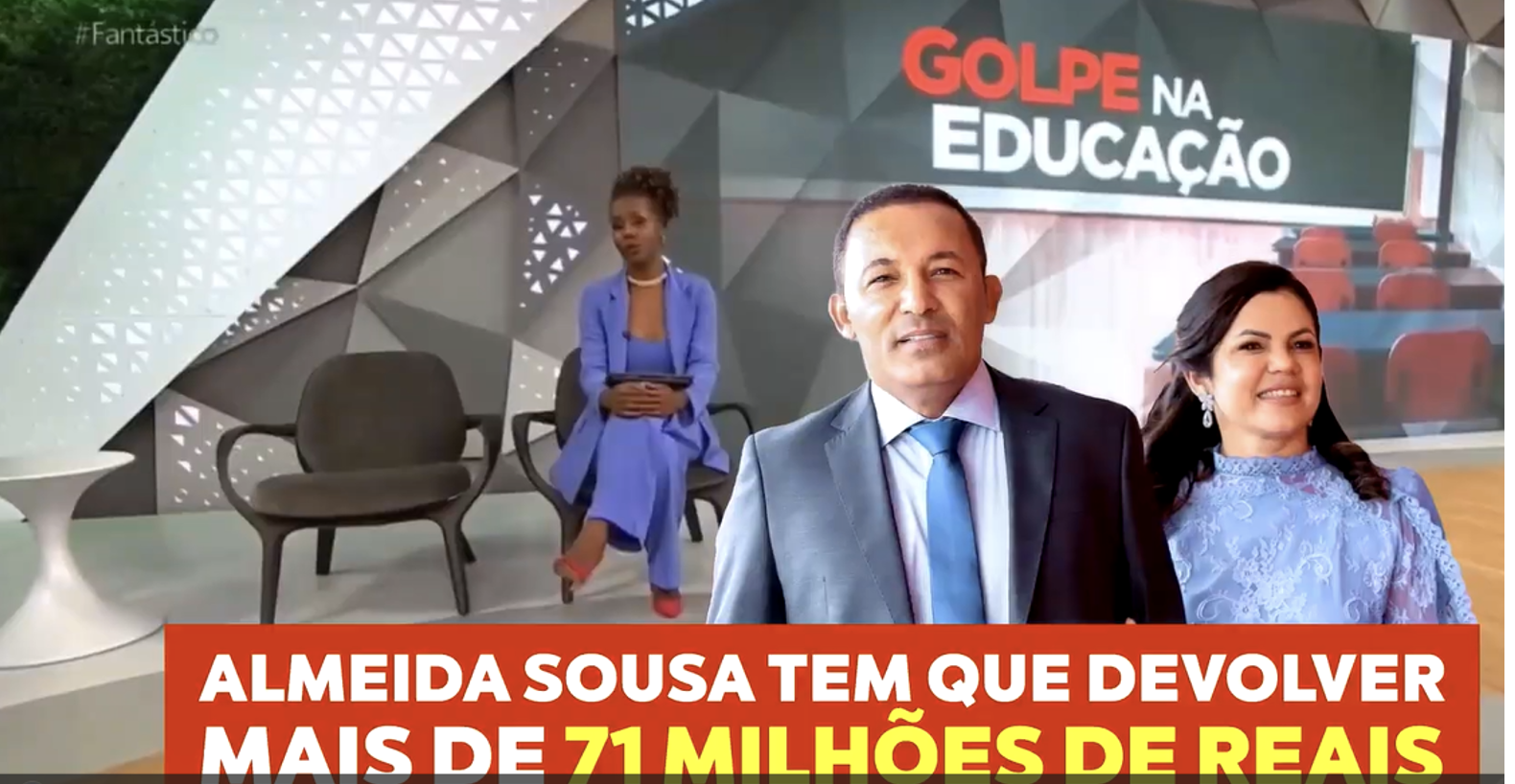
Looking forward
It is not yet clear how AI-generated visual content is received by users, especially with limited cases resulting in a breakthrough. Nevertheless, these emerging cases and previous research raise concerns that malign actors now have cheap tools that can speed up the creation of a variety of tailor-made and targeted media content to support influence operations. While various technical and non-technical verification methods used by researchers evolve in parallel, and the increased quality produced by AI tools does not guarantee credibility, this trajectory shows a worrying future trend in which the volume of disinformation outpaces detection frameworks.
Editor’s note: Ali Chenrose is a pen name used by the DFRLab for personal safety reasons.
Cite this case study:
Ali Chenrose and Max Rizzuto, “The evolving role of AI-generated media in shaping disinformation campaigns,” Digital Forensic Research Lab (DFRLab), May 1, 2025, https://dfrlab.org/2025/04/25/the-evolving-role-of-ai-generated-media-in-shaping-disinformation-campaigns/.